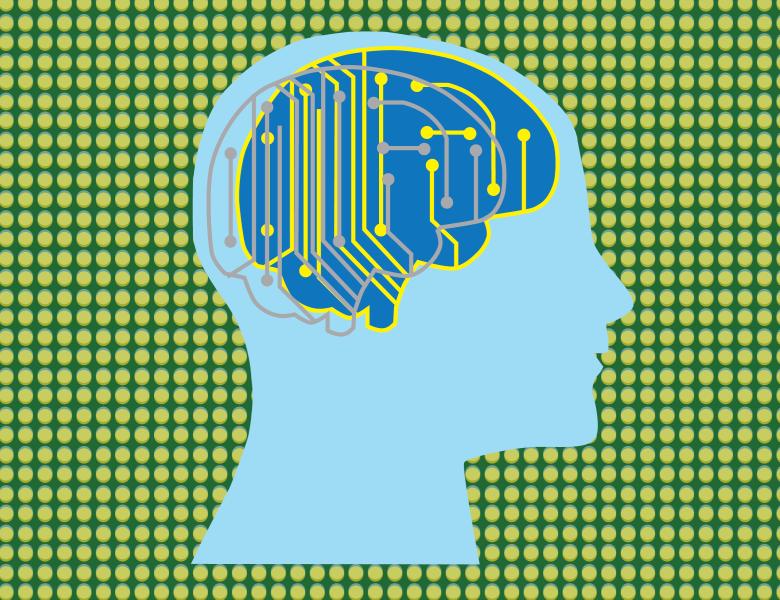
Abstract
A neural system can flexibly perform many tasks, the underlying mechanism is unknown. Here, we trained a single recurrent network model, using a machine learning method, to perform 20 cognitive tasks that may involve working memory, decision-making, categorization and inhibitory control. We found that after training the emerging task representations are organized in the form of clustering of recurrent units. Moreover, we introduce a measure to quantify single-unit neural relationships between 190 pairs of tasks, and report five distinct types of such relationship that can be tested experimentally. Surprisingly, our network developed compositionality of task representations, a critical feature for cognitive flexibility, thereby one task can be instructed by combining representations for other tasks. Finally, we demonstrate how the network could learn multiple tasks sequentially. This work provides a computational platform to investigate neural representations of many cognitive tasks, and suggests new research directions at the interface between neuroscience and AI.