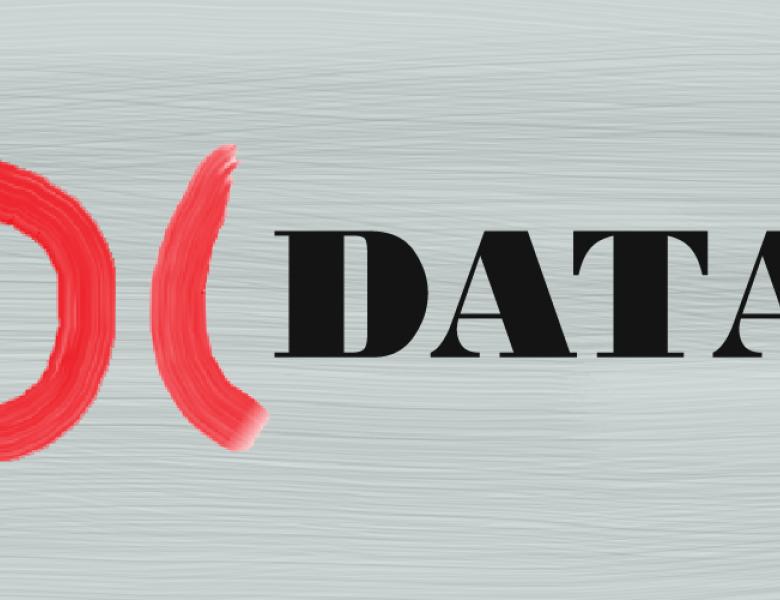
Abstract
This talk discusses a general technique to lower bound Bayes risks for arbitrary prior distributions and loss functions. A lower bound on the Bayes risk not only serves as a lower bound on the minimax risk but also characterizes the fundamental limitations of the statistical difficulty of a decision problem under a given prior.
In our method, the key quantity for computing Bayes risk lower bound is the $f$-informativity. We derive a new upper bound of $f$-informativity for a class of $f$ functions, which lead to tight Bayes risk lower bounds. We present Bayes risk lower bounds for several estimation problems as examples, including Gaussian location models, Bayesian Lasso, Bayesian generalized linear model and principle component analysis for spiked covariance model. Our technique also leads to generalizations of a variety of classical minimax bounds. In particular, our result gives the most general Fano's inequality under an arbitrary prior distribution over the parameter space. In addition to Kullback-Leibler divergence based Fano's inequality, our technique leads to a suite of lower bounds on testing risks with different $f$-divergence such as chi-squared, total variation and Hellinger distance. Further, classical results in minimax theory including Le Cam, Assouad and Birge's inequality can all be easily derived by our method.