Image
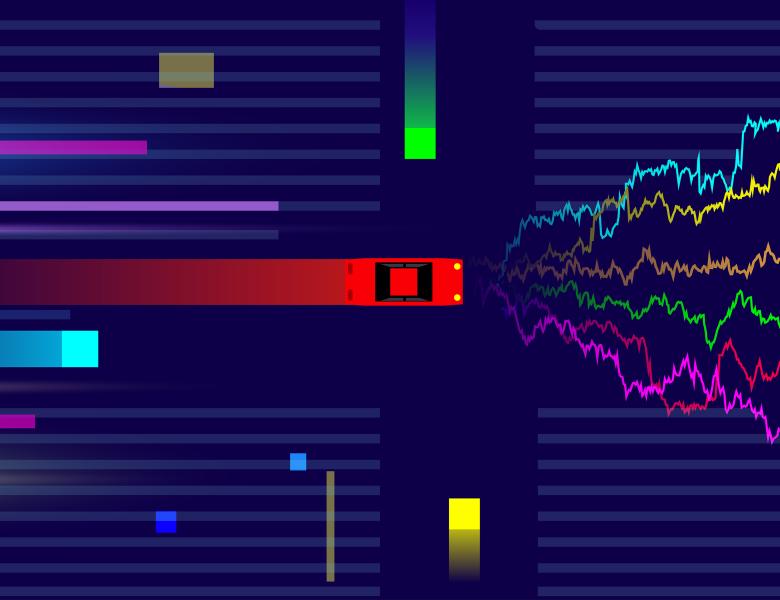
We derive learning-theoretic guarantees for inventory policies that are trained offline on a collection of (uncensored) demand sequences. We consider the periodic review of a single durable good over a finite time horizon. We show that base stock policies have normalized generalization and estimation errors that are invariant in the length of the time horizon, even if the base stocks change with time. In stark contrast, we show that (s,S) policies have errors that grow logarithmically in the length of the time horizon, even if the parameters (s,S) are fixed over time. Based on preliminary work with Yaqi Xie and Linwei Xin from Chicago Booth.