Image
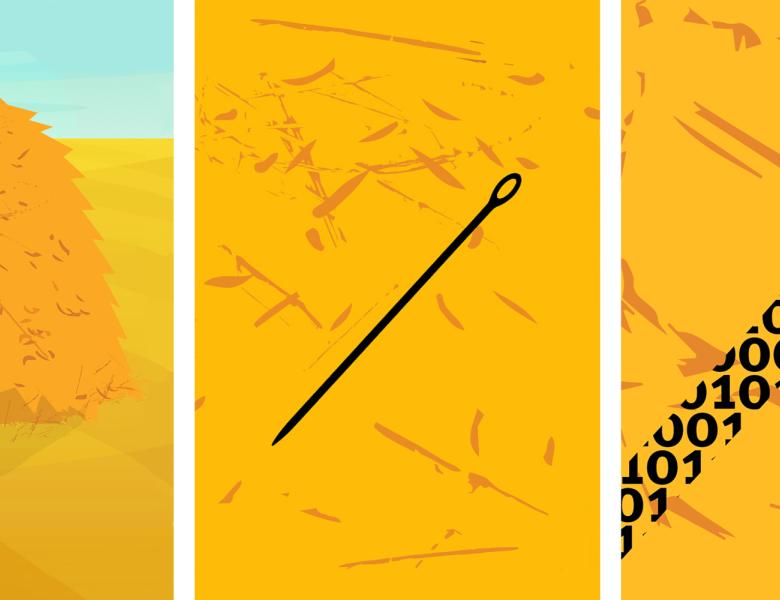
While the K-SAT problem is the cornerstone of the worst-case computational complexity theory, the random K-SAT problem serves for decades as a prototype of probability distribution to explore the average computational hardness of natural distributions of instances. While a complete and formal theory is not yet in sight, progress has been made in various directions. In this talk, I will review what we know about the algorithmic hardness of random K-SAT and more generally random constraint satisfaction problems. Several concrete conjectures about where the really hard problems really are will be discussed. I will also present close connections to the hardness of problems in statistical inference and learning.