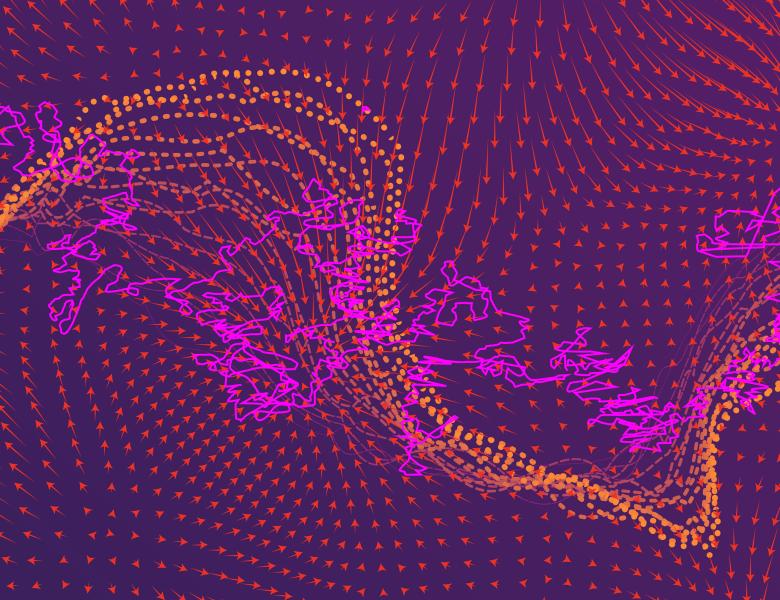
Abstract
There has been a surge of works bridging MCMC sampling and optimization, with a specific focus on translating non-asymptotic convergence guarantees for optimization problems into the analysis of Langevin algorithms in MCMC sampling. A conspicuous distinction between the convergence analysis of Langevin sampling and that of optimization is that all known convergence rates for Langevin algorithms depend on the dimensionality of the problem, whereas the convergence rates for optimization are dimension-free for convex problems. Whether a dimension independent convergence rate can be achieved by Langevin algorithm is thus a long-standing open problem. In this talk, I will discuss a composite optimization approach that leads to dimension independent convergence rates for a wide class of problems.