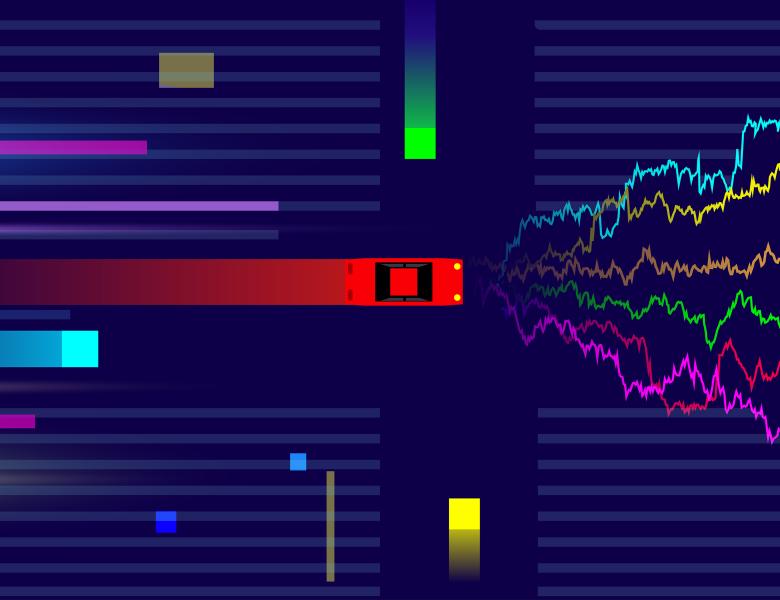
Abstract
We consider the infinite-horizon restless bandit problem with the average reward criterion. A fundamental question is how to design computationally efficient policies that achieve a diminishing optimality gap as the number of arms, N, grows large. Existing results on asymptotical optimality all rely on the uniform global attractor property (UGAP), a complex and challenging-to-verify assumption. In this talk, we discuss how this assumption can be removed. We present various new algorithms that unveil more insights on how to achieve asymptotic optimality.