Image
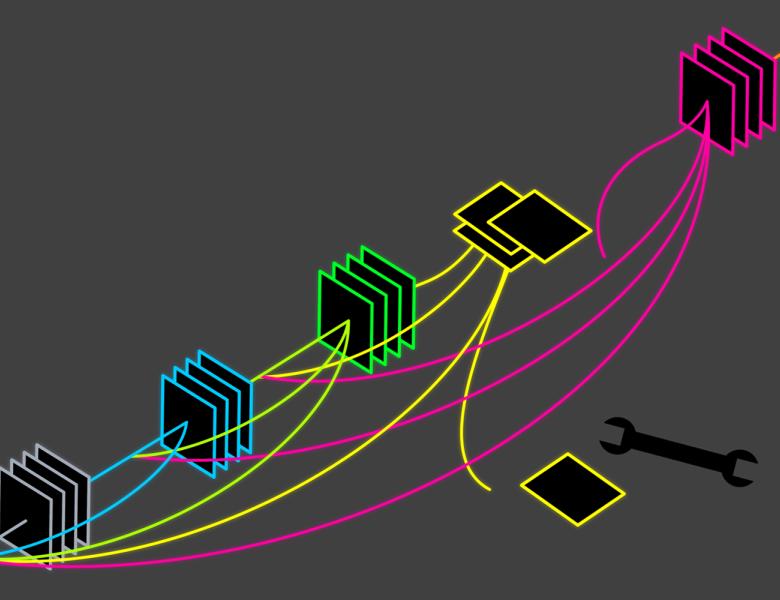
Can uniform convergence explain interpolation learning? In our struggle to understand interpolation learning, we have mostly dismissed the classical statistical learning theory approach based on uniform convergence. But interpolation learning in some, or perhaps all, regimes be understood using refined enough uniform convergence? I recent work with Lijia Zhou and Dougal Southerland we show that at least in a simple noise undetermined linear model uniform convergence, when done carefully and in a risk-dependent manner, might in fact be sufficient for analyzing interpolation learning.