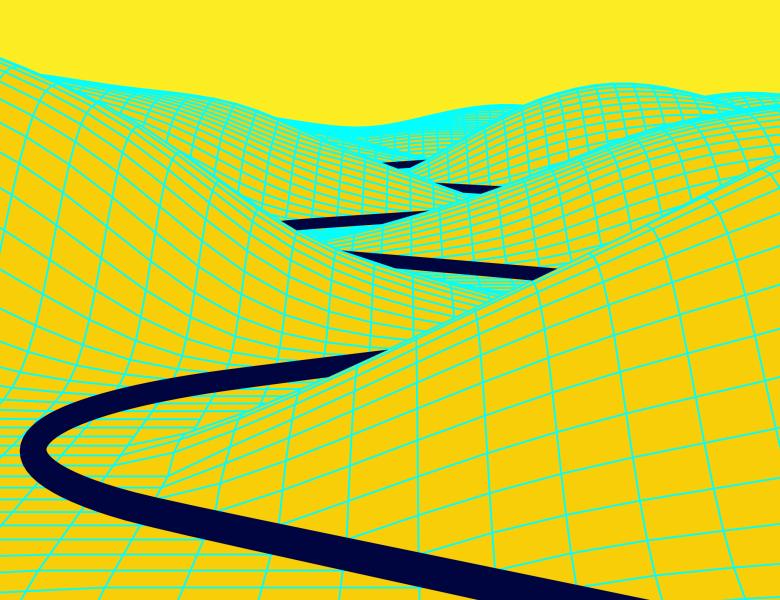
Abstract
Abstract: Deep networks often fail catastrophically under the presence of distribution shift—when the test distribution differs in some systematic way from the training distribution. Robustness to distribution shifts is typically studied for its crucial role in reliable real-world deployment of deep networks. In this talk, we will see that robustness can also provide new insights into the functioning of deep networks, beyond the standard generalization puzzle. First, we will dive into the popular setting of transferring a pre-trained model to a downstream task. We study the optimization dynamics of the transfer process in a stylized setting that replicates observed empirical observations and allows us to devise a new heuristic that outperforms previous methods. Next, we will go over several observations from robustness in the standard supervised setting that provide a new perspective on the role of overparameterization and the inductive biases of deep networks.