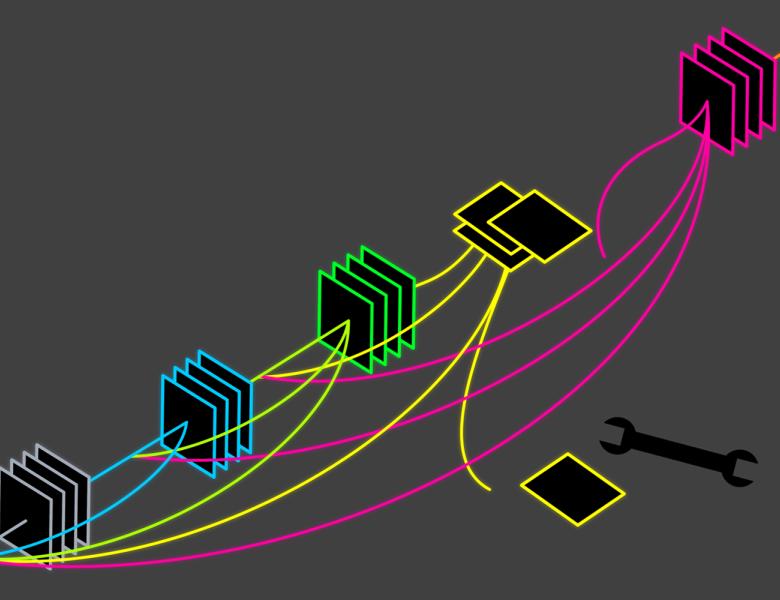
Abstract
This talk will briefly illustrate two properties of neural networks: firstly, the parameters found by gradient descent asymptotically converge and satisfy a certain alignment property, which is assumed throughout the implicit regularization literature and has further implications there; secondly, good NTK-style behavior near initialization does not always need width proportional to the number of examples, and instead can be described by the NTK margin, which can be much smaller. Joint work with Ziwei Ji.