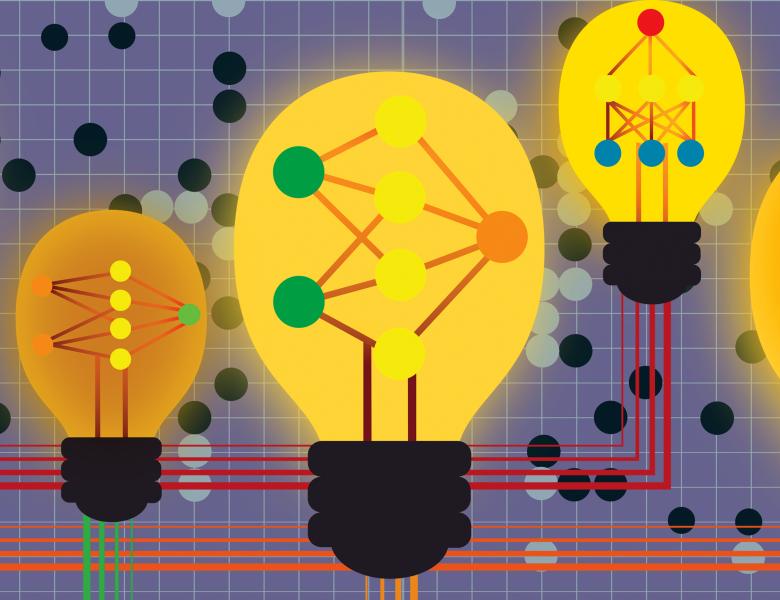
Abstract
Title: The Transfer Performance of Economic Models" (joint with Isaiah Andrews, Drew Fudenberg, and Chaofeng Wu)
Abstract: Economists often estimate models using data from a particular setting, e.g. estimating risk preferences in a specific subject pool. Whether a model's predictions extrapolate well across settings depends on whether the model has learned generalizable structure. We provide a tractable formulation for this "out-of-domain" prediction problem, and define the transfer error of a model to be its performance on data from a new domain. We derive finite-sample forecast intervals that are guaranteed to cover realized transfer errors with a user-selected probability when domains are drawn iid from some population, and apply our approach to compare the transferability of economic models and black box algorithms for predicting certainty equivalents. While the black box algorithms outperform the economic models in traditional "within-domain" tests (i.e., when estimated and tested on data from the same domain), in this application models motivated by economic theory transfer more reliably than black-box machine learning methods do.