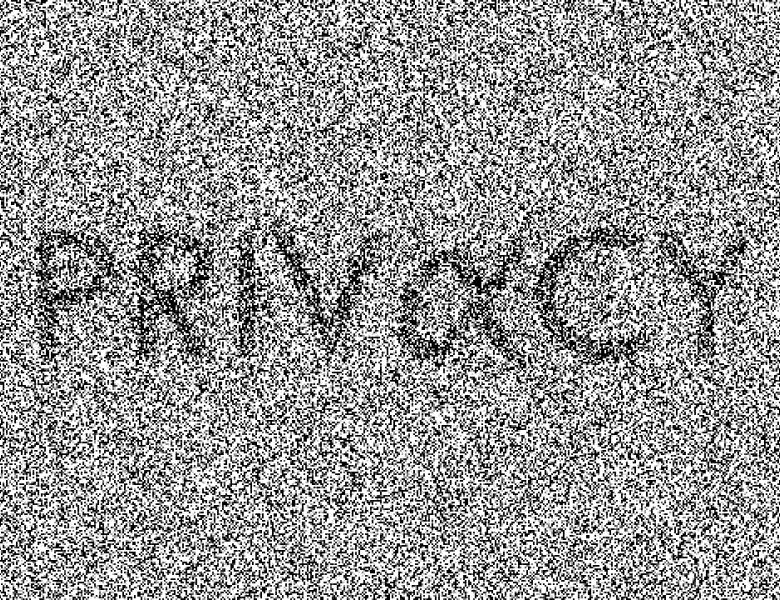
Abstract
In this talk, we investigate the role of information in *fair* prediction. A common strategy for decision-making uses a predictor to assign individuals a risk score; then, individuals are selected or rejected on the basis of this score. We formalize a framework for measuring the information content of such predictors, showing that increasing information content through a certain kind of "refinement" improves the downstream selection rules across a wide range of fairness measures (e.g. true positive rates, false positive rates, selection rates). In turn, refinements provide a simple but effective tool for reducing disparity in treatment and impact without sacrificing the utility of the predictions. Our results suggest that in many applications, the perceived “cost of fairness” results from an information disparity across populations, and thus, may be avoided with improved information. We conclude by discussing how our information-theoretic perspective on fairness may shed new light on the feasibility of simultaneously *fair and private* predictions. Based on joint work with Sumegha Garg and Omer Reingold.