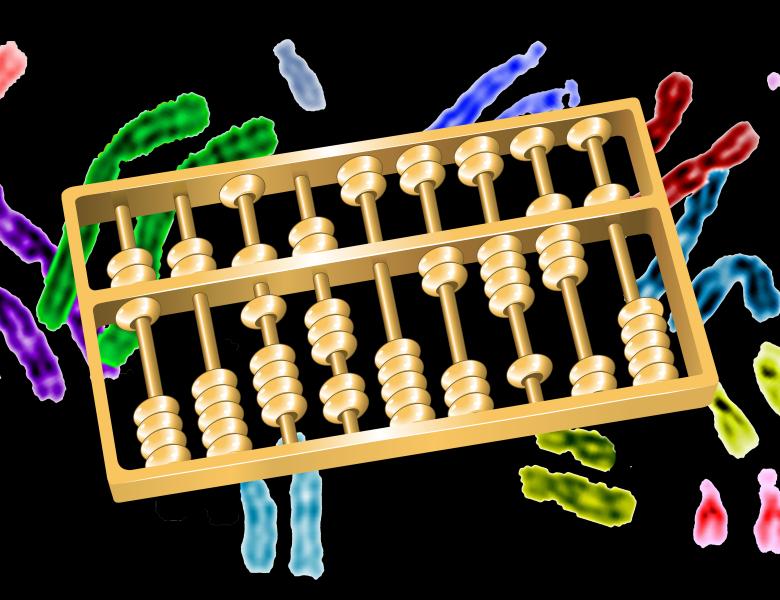
Abstract
High-throughput data that are being collected on tumor samples create a massive opportunity to uncover the inner workings of tumor cells, how they interact with tissue microenvironments, and why they respond or resist treatments. While we have gleaned a great deal of insight from such troves of data and have made important inroads, several challenges remain. First, sequencing projects continue to produce huge lists of events (mutations, deletions, fusions, etc) of unknown significance. Methods for integrating and interpreting the findings using genetic pathway information will be discussed.
Second, the collection of integrated data and the formation of related patient subtypes from these data has uncovered new connections both within and across tumor subtypes. The tissue-of-origin of a tumor has been shown to be the strongest signal in nearly all platforms of data. However, several cross-tumor connections have already been identified with important implications for patient outcomes that I will present. New methods are needed to look beyond the tissue to probe other important aspects of tumor biology including the initiating and driving oncogenic processes, the original cell type that was transformed by the genetic assaults, and the impacted genetic pathways that might provide clues about treatment. I’ll discuss an approach to identify differentiation and de-differentiation status in minor but important fractions of tumor samples that may provide key information for understanding response. Rather than use out-of-the-box machine-learning methods for training signatures, I’ll discuss the development of biologically-motivated methods that seek solutions that match our current understanding of genetic circuitry.
Finally, the dearth of data should inform the biology of each patient individually, however very little tools exist for leveraging large compendia for an individual patient’s use. Each patient can have a unique combination of genetic alterations, gene expression changes, hypo- or hyper-methylated enhancers, and so on. At the same time, we can often identify a set of samples that share common properties (e.g. mRNA or miRNA transcriptional profiles) with a particular patient of interest. The question remains how best to use a comprehensive collection of data to maximally enlighten our understanding of even one tumor sample. Clearly, using the statistical power of the large collection is necessary to bring robustness and confidence to the investigation. On the other hand, ignoring patient- and specimen-specific properties (e.g. newly acquired mutations after treatment) could over-generalize and miss important differences in a particular patient, not shared by the many, that could uncover druggable opportunities not otherwise apparent. Thus, new computational methods are needed that strike the right balance for interpreting each “N-of-1” case. I’ll discuss approaches we are developing for visualizing and inferring patient-specific networks.