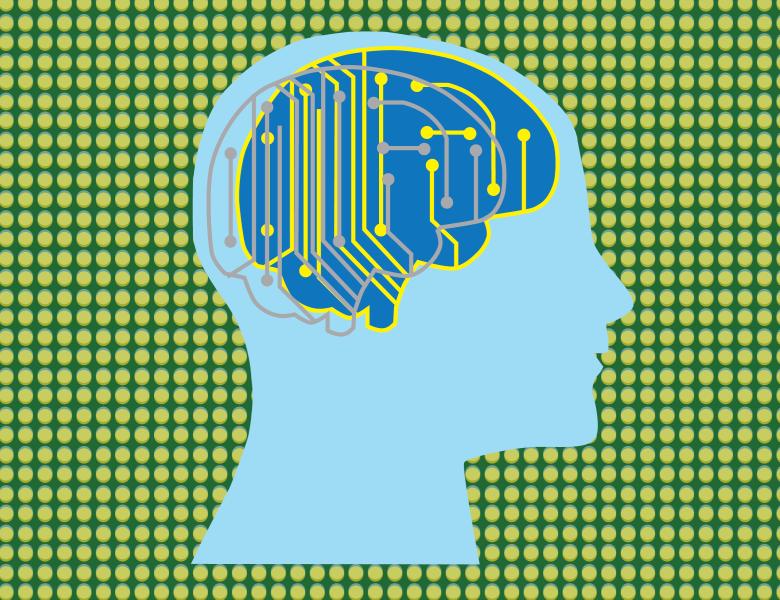
Abstract
There has been rapid progress in the application of machine learning to difficult problems such as: voice and image recognition, playing video games from raw-pixels, controlling high-dimensional motor systems, and winning at the games of Go, Chess and Poker. These recent advances have been made possible by employing the backpropagation-of-error algorithm. This algorithm enables the delivery of detailed error feedback to adjust synaptic weights, which means that even large networks can be effectively trained. Whether or not the brain employs similar deep learning algorithms remains contentious; how it might do so remains a mystery. I will begin by reviewing advances in deep reinforcement learning that highlight the importance of backprop for effectively learning complex behaviours. Then I will describe recent neuroscience evidence that suggests an increasingly complex picture of the neuron. This picture emphasizes the importance of electrotonically segregated compartments and the computational role of dendrites. Taken together, these findings suggest new ways that deep learning algorithms might be implemented in cortical networks in the brain.