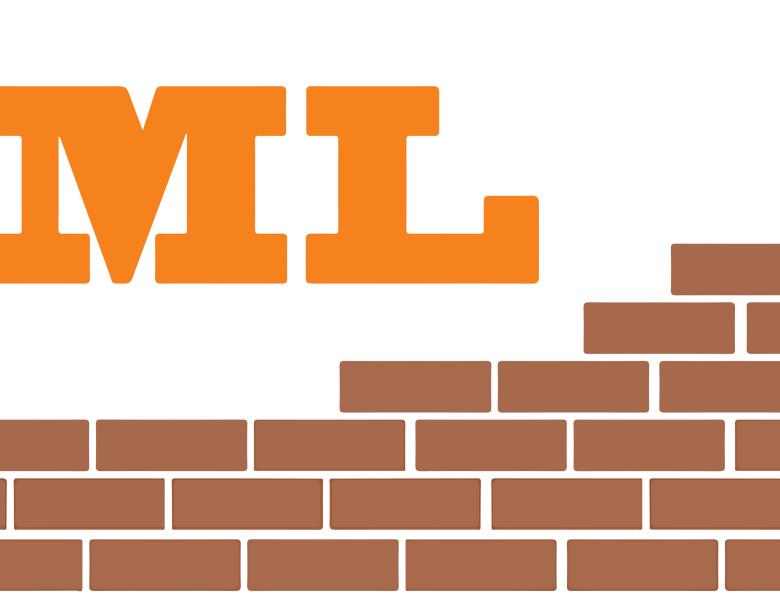
Abstract
In this talk, I will present a new general active learning strategy, yielding significantly better label complexity guarantees than previously known to be possible. In particular, this work reveals that under Tsybakov's noise condition, the minimax optimal label complexity of active learning is significantly smaller than that of traditional (passive) supervised learning, for any hypothesis class of finite VC dimension. This new active learning strategy essentially uses sequential hypothesis tests, modified with an early cut-off stopping criterion, to enhance the noise-robustness of a simpler active learning subroutine. The method is surprisingly simple, and quite general, and also yields near-optimal label complexities in several other settings, such as learning with a smooth regression function or a smooth decision boundary.