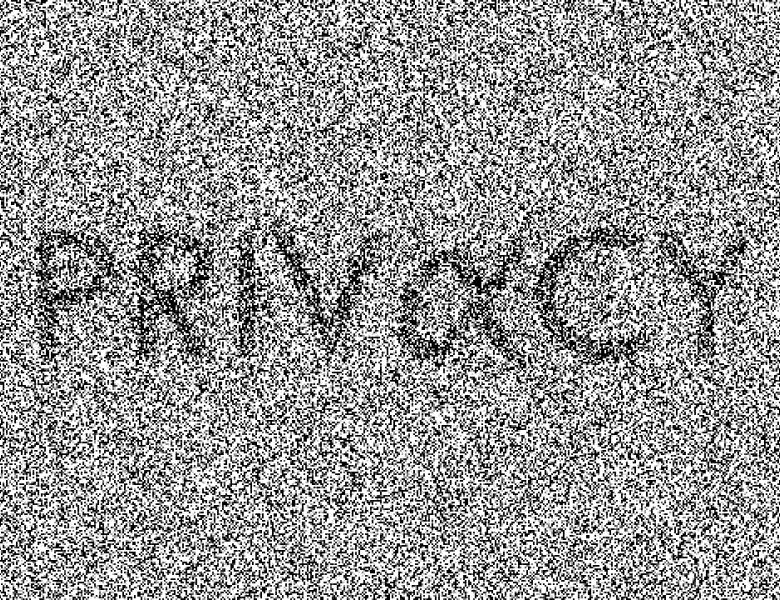
Abstract
Since its inception as a field of study roughly one decade ago, research in algorithmic fairness has exploded. Much of this work focuses on so-called "group fairness" notions, which address the relative treatment of different demographic groups. More theoretical work advocated for "individual fairness" which, speaking intuitively, requires that people who are similar, with respect to a given classification task, should be treated similarly by classifiers for that task. Both approaches face significant challenges: for example, provable incompatibility of natural fairness desiderata (for the group case), and the absence of similarity information (for the individual case).
In the last two years the theory literature has begun to explore notions that try to bridge the gap between group and individual fairness. These works raise compelling questions with ties to well-studied topics in statistics, such as forecasting and combining the opinions of experts, as well as psychology, law, economics, and political science. We will discuss some of these questions from the starting point of multi-calibration (Hebert-Johnson, Kim, Reingold, and Rothblum, 2018) and very recent related work on evidence-based rankings (Dwork, Kim, Reingold, Rothblum, and Yona).