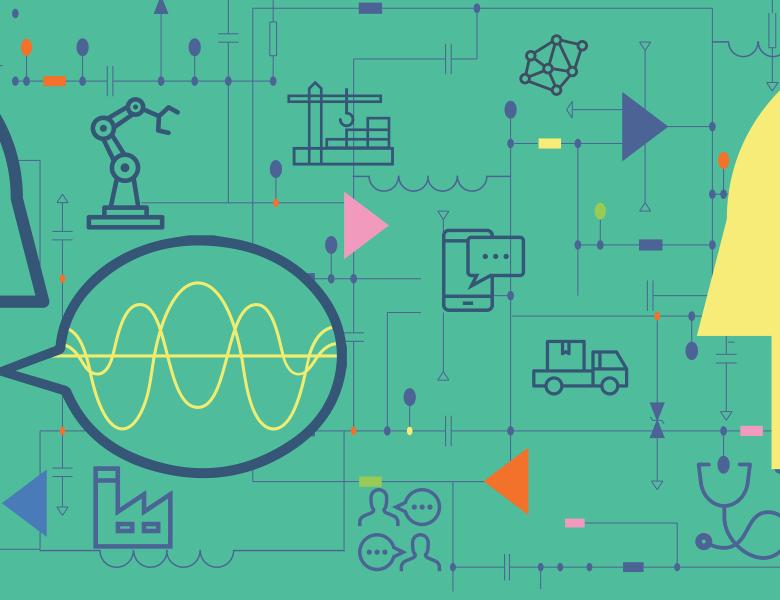
Abstract
Robust control theory highlighted the importance of quantifying model uncertainty for the design of feedback control strategies that achieve a provable level of performance. The robustness paradigm motivated work on ‘robust learning’ to address the question of how well model uncertainty can be characterized from data. The quality and convergence rate of model approximation from data imposes a fundamental limit on the rate of adaptation of the underlying control/decision strategy. In particular, for some model classes, sample complexity results impose significant limitations on such adaptation rates. We conjecture that the same limitations exist for convergence in reinforcement learning.
The characterization of the relationship between learning and model uncertainty hinges on having a tractable theory for model approximation. Such a theory exists for broad classes of linear stochastic dynamical systems. In this talk, I will present some results for learning classes of stochastic systems, namely, jump linear systems. A key question for such models is the unraveling of the underlying model structure from data. In this context, I will show that spectral methods allow for estimating both the underlying state dimension when the number of stochastic transitions is known. Utilizing existing results from model reduction using Hankel-like matrices, I will show that efficient learning of low dimensional models is quite possible.
This work is in collaboration with Tuhin Sarkar and Alexander Rakhlin.