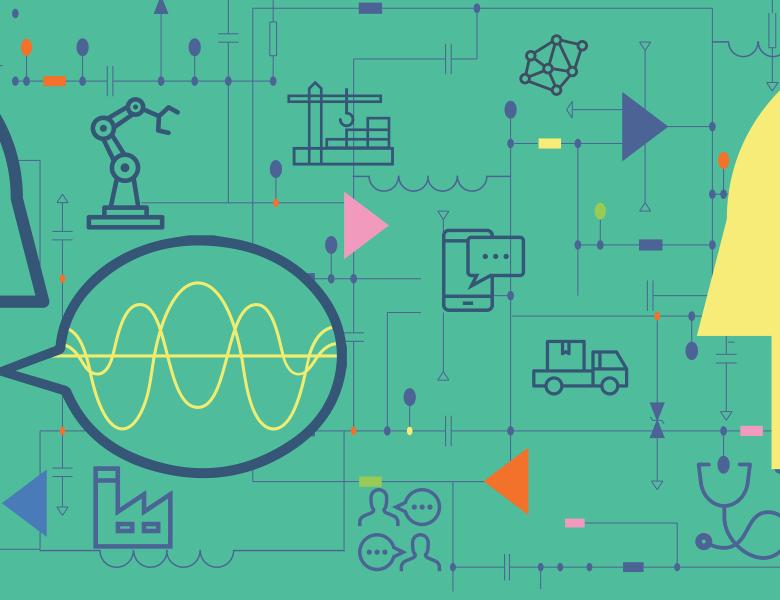
Abstract
It is often the case that the guarantees provided by worst-case analysis can be overly pessimistic, lessening their utility to the practitioner. Instance-dependent bounds allow one to formalize the notion that "not all problems are equal"---some are easier than others. As one foray in this general area, we focus on the problem of policy evaluation in tabular MDPs, asking whether or not the classical TD algorithm is optimal. We first prove local minimax lower bounds on the problem, which reveals the local features that control the difficulty of the problem. We then show that that standard TD-learning, even when combined with Polyak-Ruppert averaging, is not an instance-optimal procedure. Finally, we describe a simple variant of TD-learning, and establish its instance-optimaltiy.
Based on joint work with Koulik Khamaru, Ashwin Pananjady, Feng Ruan and Michael Jordan.