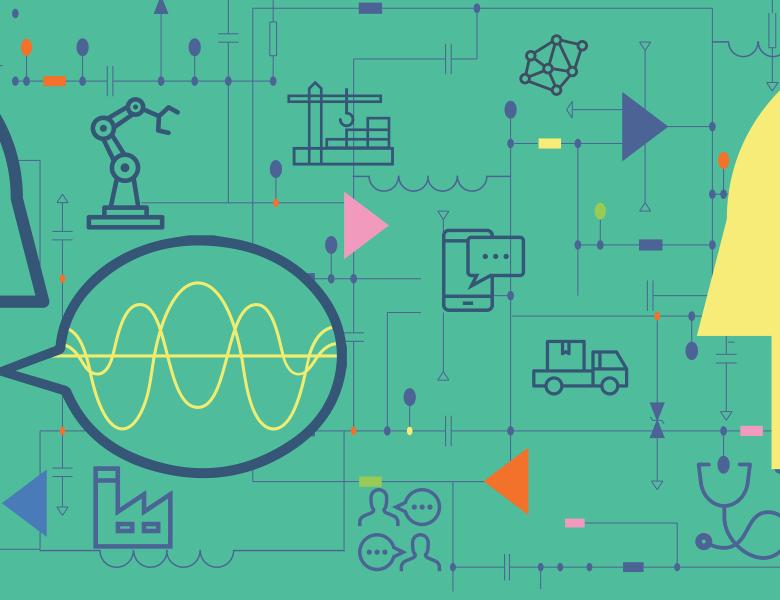
Abstract
Many estimands of interest in sequential decision problems are non-smooth functionals of the data-generating distribution. Examples include the marginal mean outcome under an optimal policy and coefficients indexing regression models in approximate dynamic programming. We review how this non-regularity induces instability which in turn can cause standard inference procedures based on asymptotic approximations to perform poorly. We derive inference procedures based on bounding a non-regular functional between two smooth functionals and show that the resulting inference is valid under fixed and moving parameter asymptotic frameworks. We also show that in some cases, the resulting procedures are universal (i.e., provide consistent coverage uniformly over a large class of distributions) and also provide conditional coverage. Having derived confidence intervals and tests for the estimands of interest, we then consider power and sample size calculations based on these estimands.