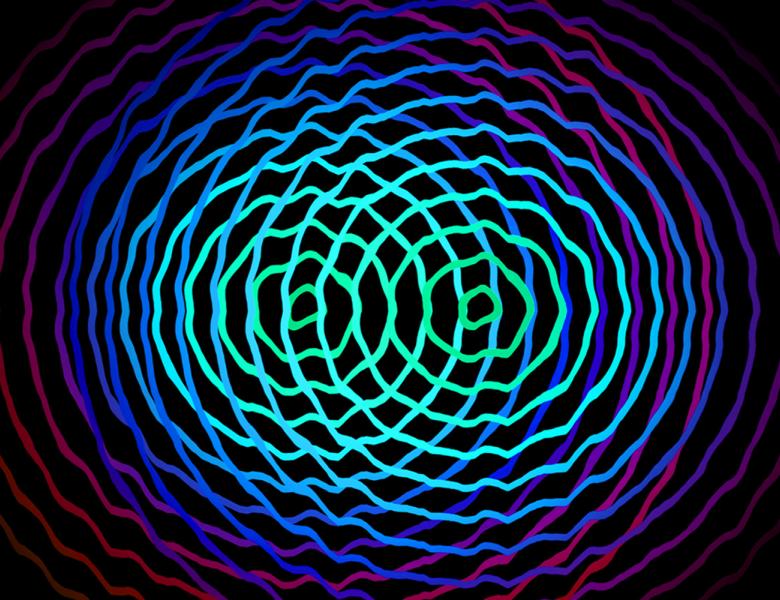
Abstract
Variational Quantum Circuits, which include examples of quantum approximate optimization algorithms (QAOA), variational quantum eigensolver (VQE), and quantum neural-networks (QNN), are predicted to be one of the most important near-term quantum applications, not only because of their potential promises as classical neural-networks but also because of their feasibility on near-term noisy intermediate size quantum (NISQ) machines.
This talk reports some progress toward a principled understanding of the training of variational quantum circuits. First, I will demonstrate the difficulty of training by constructing an example with exponentially many local optima, however, due to a differential nature from classical neural-networks. Second, I will explain how to facilitate the training by incorporating the optimal-transport norm in the context of quantum generative adversarial networks (GANs), as well as its application in compressing quantum circuits in practical uses.