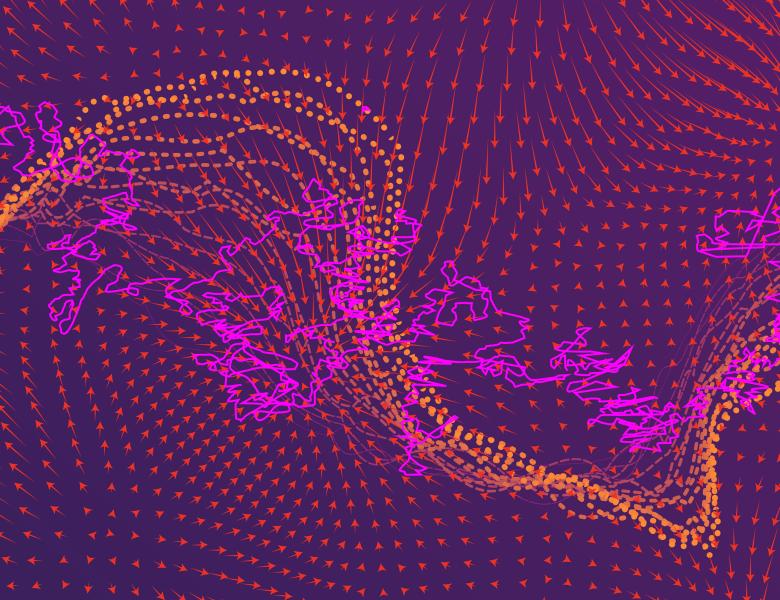
Abstract
We survey two recent developments at the frontier of differentially private convex optimization, which draw heavily upon geometric tools in optimization and sampling.
The first result proposes a new framework for differentially private optimization of convex functions which are Lipschitz in an arbitrary norm, based on a regularized exponential mechanism, generalizing a recent work of Gopi, Lee, and Liu '22 to non-Euclidean settings. We show that this mechanism satisfies Gaussian differential privacy and solves both DP-ERM (empirical risk minimization) and DP-SCO (stochastic convex optimization) by using localization tools from convex geometry. Our framework is the first to apply to private convex optimization in general normed spaces and directly recovers non-private SCO rates achieved by mirror descent as the privacy parameter ϵ→∞. For Lipschitz optimization in ℓp norms and Schatten-p norms for all p∈(1,2), we obtain the first optimal privacy-utility tradeoffs.
The second result introduces a new tool for stochastic convex optimization (SCO): a Reweighted Stochastic Query (ReSQue) estimator for the gradient of a function convolved with a (Gaussian) probability density. Combining ReSQue with recent advances in "ball oracle acceleration" (Carmon et al '20, Asi et al '21), we develop algorithms achieving state-of-the-art complexities for DP-SCO. We give an (ϵ, 𝛿)-DP algorithm which, given n samples of Lipschitz loss functions, obtains near-optimal optimization error and makes min(n, n^2 ϵ^2 d^{-1}) + \min(n^{4/3} ϵ^{1/3}, (nd)^{2/3} ϵ^{-1})$ queries to function gradients. This improves recent advancements of Kulkarni et al '21, Asi et al '21, and is near-linear in moderately low-dimensional settings.
Based on joint works with Yair Carmon, Sivakanth Gopi, Arun Jambulapati, Yujia Jin, Yin Tat Lee, Daogao Liu, Ruoqi Shen, and Aaron Sidford.