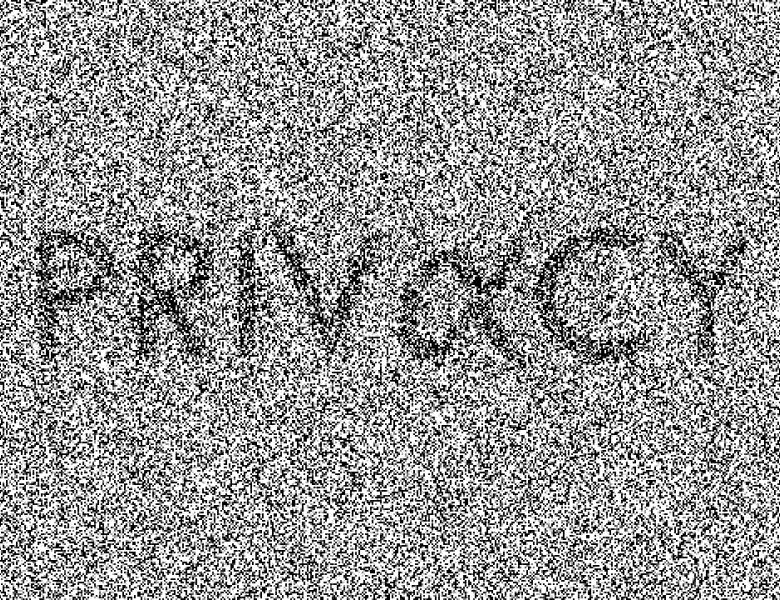
Abstract
This talk will start with an overview of the traditional statistical disclosure limitation (SDL) framework implemented at statistical agencies for standard outputs, including types of disclosure risks, how disclosure risk and information loss are quantified, and some common SDL methods. Traditional SDL approaches were developed to protect against the risk of re-identification which is grounded in legislation and statistics acts. In recent years, however, we have seen the digitalization of all aspects of our society leading to new and linked data sources offering unprecedented opportunities for research and evidence-based policies. These developments have put pressure on statistical agencies to provide broader and more open access to their data. On the other hand, with detailed personal information easily accessible from the internet, traditional SDL methods for protecting individuals may no longer be sufficient and this has led to agencies relying more on restricting and licensing data. With increasing demands for more open and accessible data, the disclosure risk of concern for statistical agencies has shifted from the risk of re-identification to inferential disclosure where confidential information may be revealed exactly or to a close approximation. Statistical agencies are now revisiting their intruder scenarios and types of disclosure risks and assessing new privacy models with more rigorous data protection (perturbative) mechanisms for more open strategies of dissemination. Statisticians are now investigating the possibilities of incorporating Differential Privacy (Dwork, et al 2006) into their SDL framework, especially for web-based dissemination applications where outputs are generated and protected on-the-fly without the need for human intervention to check for disclosure risks. We discuss these dissemination strategies and the potential for Differential Privacy to provide privacy guarantees against inferential disclosure.