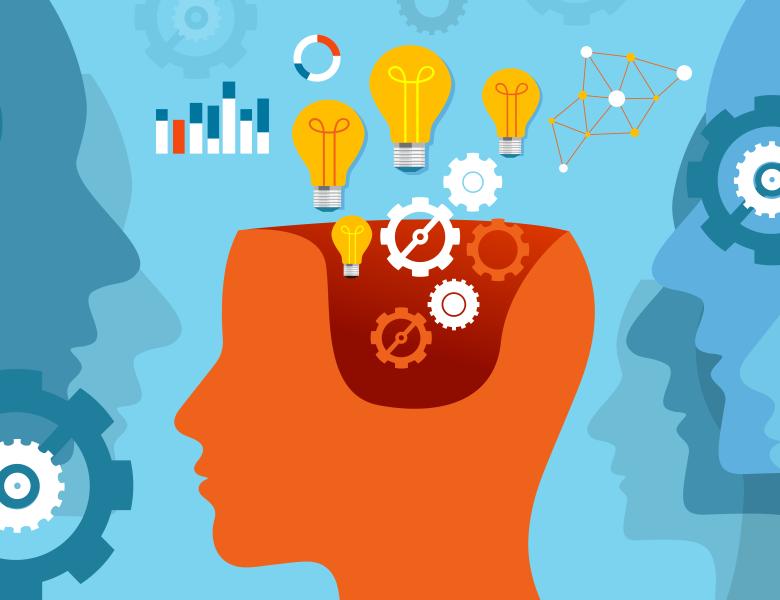
Abstract
This talk provides a new theoretical framework to explain the success of contrastive pre-training in downstream tasks, such as zero-shot classification, conditional diffusion models, and vision-language models. We introduce the concept of approximate sufficient statistics, a generalization of the classical sufficient statistics, and show that near-minimizers of the contrastive pre-training loss are approximately sufficient, making them adaptable to diverse downstream tasks. We further propose the Joint Generative Hierarchical Model for the joint distribution of images and text, showing that transformers can efficiently approximate relevant functions within this model via belief propagation. Building on this framework, we derive sample complexity guarantees for multi-modal learning based on contrastive pre-trained representations, validated through numerical simulations.
This is joint work with Kazusato Oko, Licong Lin, and Yuhang Cai. arXiv Preprint: https://arxiv.org/abs/2501.04641