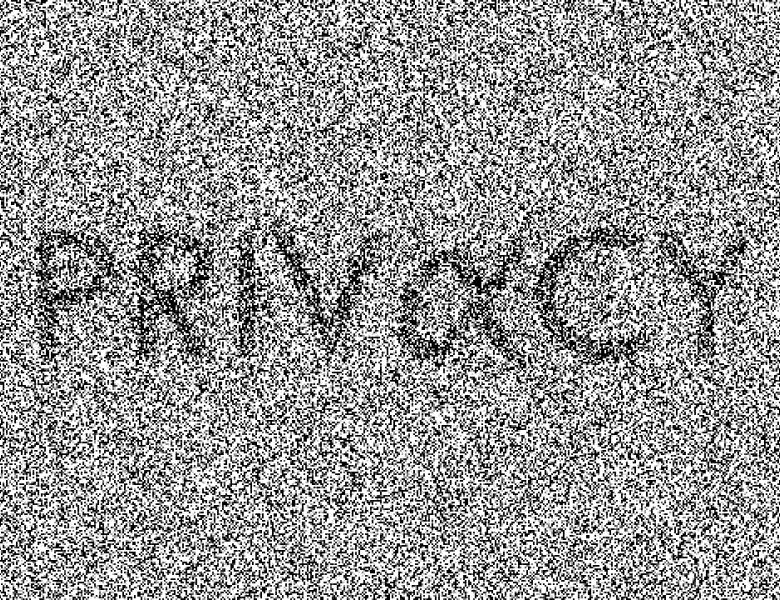
Abstract
Noise addition is the most basic technique for guaranteeing differential privacy. However, usually noise is scaled to the "worst-case" or "global" sensitivity of the function being computed and significant efforts are taken to ensure that this is minimized. Nissim, Raskhodnikova, & Smith (STOC 2007) showed that it is possible to add substantially less noise provided that the function has low "local" sensitivity on the realized dataset and all nearby datasets. This talk revisits their "smooth sensitivity" framework and provides both new algorithms within the framework and considers applications to basic problems which can have infinite global sensitivity.