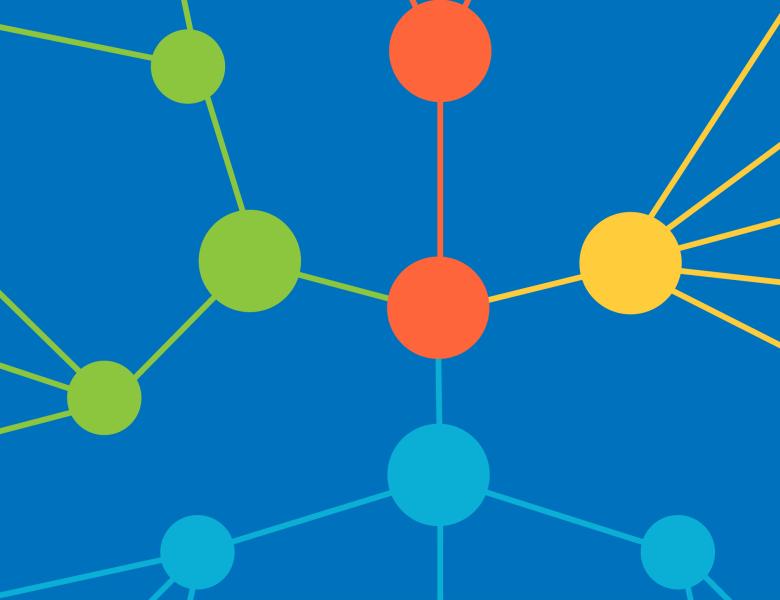
Abstract
Extrapolating scaling trends suggest that training dataset size for LLMs may soon be limited by the amount of text data available on the internet. In this talk we investigate scaling language models in data-constrained regimes. Specifically, we run a set of empirical experiments varying the extent of data repetition and compute budget. From these experiments we propose and empirically validate a scaling law for compute optimality that accounts for the decreasing value of repeated tokens and excess parameters. Finally, we discuss and experiment with approaches
for mitigating data scarcity.