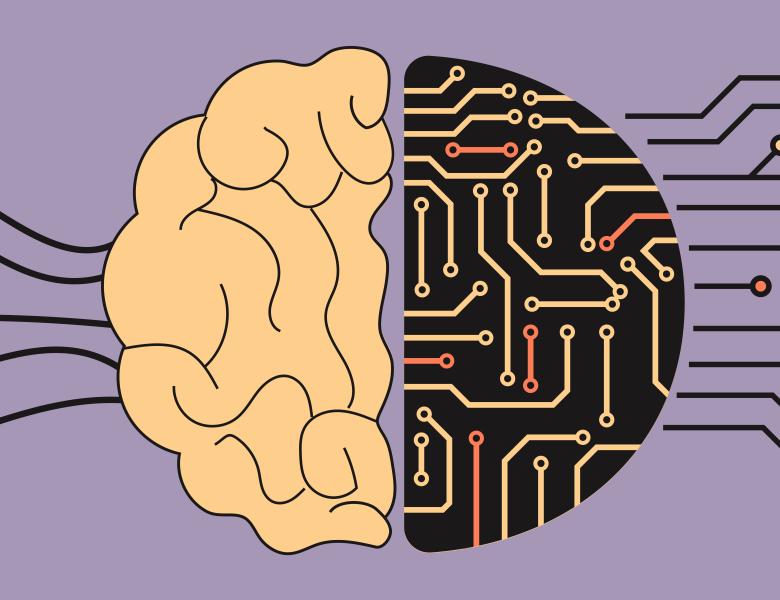
Abstract
Do Language Models understand? How can we evaluate the depth of understanding of an entity, be it human or artificial or a combination? We propose a hierarchy of understanding of algorithms, with the goal of making it precise enough to be computable. The talk will motivate and present definitions of levels of understanding along with some empirical studies with both humans and versions of GPT.
A different aspect of cognition is hallucination. Here we show a lower bound on the hallucination rate of any model calibrated to its training data, by relating it to a corresponding rate of misclassification, a classical learning theory setting; as a corollary, we obtain a measurable lower bound on the hallucination rate for "arbitrary" facts.
The talk is based on work by Mirabel Reid (Georgia Tech) and by Adam T. Kalai (OpenAI).