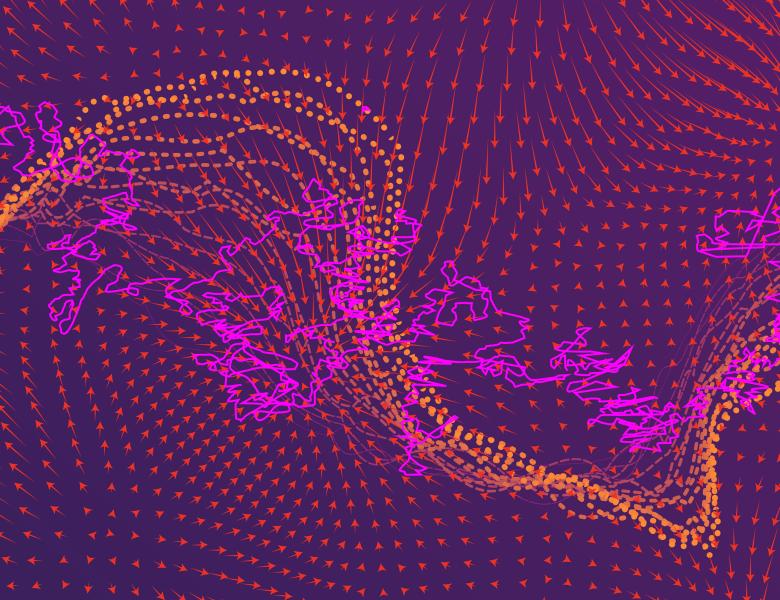
Abstract
This course introduces basic tools for the mathematical analysis of Markov Chain Monte Carlo methods.
In the first part, we review standard approaches for bounding mixing and relaxation times as well as variances of ergodic averages of Markov chains, including coupling methods, spectral gaps and conductance. We show how these approaches can be applied in simple examples to analyze basic MCMC methods such as Gibbs samplers or Metropolis-Hastings algorithms.
In the second part, we consider tools for studying the convergence to equilibrium of diffusion processes, including coupling methods and functional inequalities. We apply these to overdamped Langevin dynamics and corresponding (overdamped) Langevin algorithms. Finally, we show how degenerate noise can accelerate convergence to equilibrium, which motivates to consider (second order) Langevin dynamics and Hamiltonian Monte Carlo.