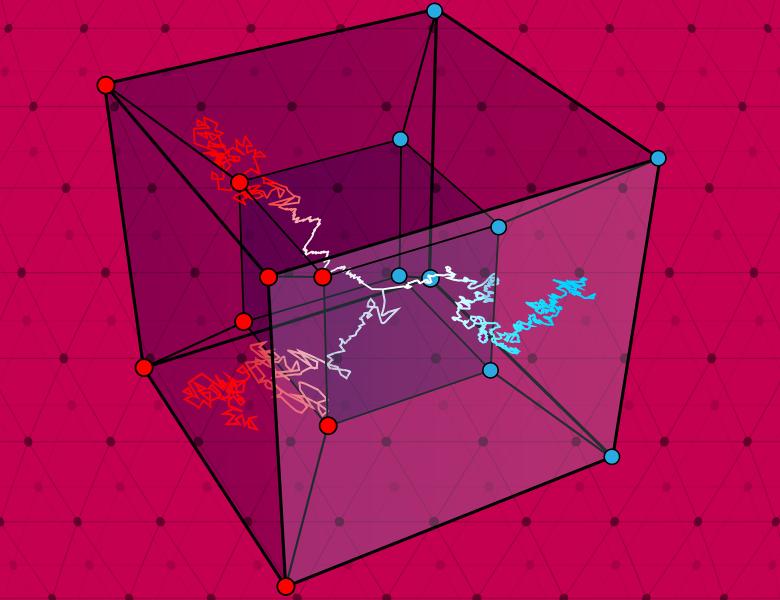
Abstract
We show that the supremum of any centered Gaussian process can be approximated to any arbitrary accuracy by a finite dimensional Gaussian process, where the dimension of the approximator is just dependent on the target error. As a corollary, we show that for any norm \Phi defined over R^n and target error \eps, there is a norm \Psi such that (i) \Psi is only dependent on t(\eps) = \exp \exp (poly(1/\eps)) dimensions and (ii) \Psi(x)/\Phi(x) \in [1-\eps, 1+ \eps] with probability 1-\eps (when x is sampled from the Gaussian space). We prove a similar-in-spirit result for sparsifying high-dimensional polytopes in Gaussian space, and present applications to computational learning and property testing. Our proof relies on Talagrand's majorizing measures theorem. Joint work with Anindya De, Shivam Nadimpalli, and Ryan O'Donnell.