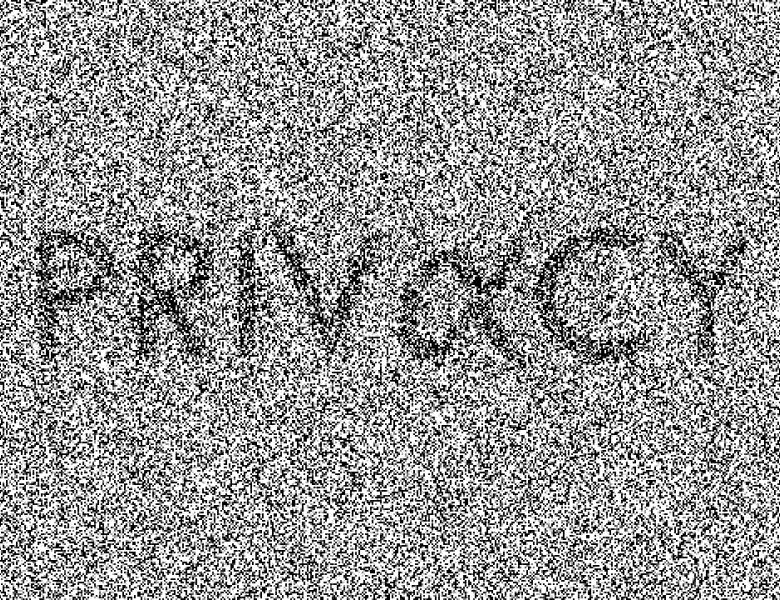
Abstract
The potential advantages of using robust statistics for privacy have already been discussed in the existing literature. However there has been little investigation on how robustness can preserve differential privacy as well as deliver statistical accuracy from the private outputs. In this talk we provide an overview of some basic elements of robust statistics that can be of relevance to privacy and additionally discuss recently proposed bias-correction (and inference) methods that can be used for this purpose. The idea we want to investigate is whether existing privacy mechanisms can be improved in terms of (statistical) utility by making use of these approaches and we study their behaviour in a simple simulation setting. The results indicate that these approaches (robustness and bias-correction) can be worth investigating further and employed more abundantly within privacy-preserving mechanisms.