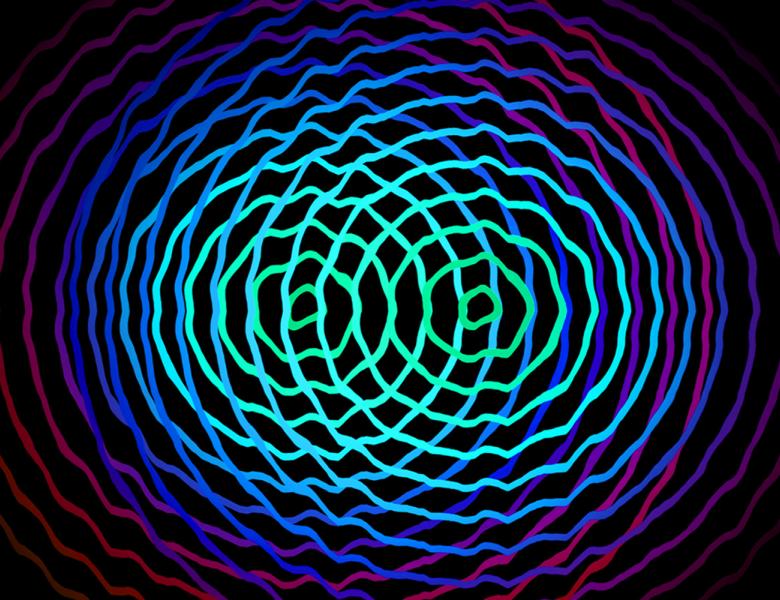
Abstract
Over the past few years several quantum machine learning algorithms were proposed that promise quantum speed-ups over their classical counterparts. Most of these learning algorithms either assume quantum access to data -- making it unclear if quantum speed-ups still exist without making these strong assumptions, or are heuristic in nature with hitherto no provable advantage over classical algorithms. Here we construct a formal learning problem and prove that the recently proposed heuristic primitive -- quantum kernel methods -- has a rigorous end-to-end quantum speed-up. The classifier is a conventional support vector machine that only requires classical access to data, where kernel entries are estimated from the transition amplitudes of a parameterized circuit family on a quantum computer. To prove the quantum speed-up, we construct a family of data sets and show that no classical learner can classify the data inverse-polynomially better than random guessing, assuming the widely-believed hardness of the discrete logarithm problem. Meanwhile, we construct a family of parameterized unitary circuits, which can be efficiently implemented on a fault tolerant quantum computer, and use them to map the data samples to a quantum feature space and estimate the kernel entries. We show that the resulting quantum classifier achieves high accuracy and is robust against additive errors in the kernel entries that arise from finite sampling statistics.