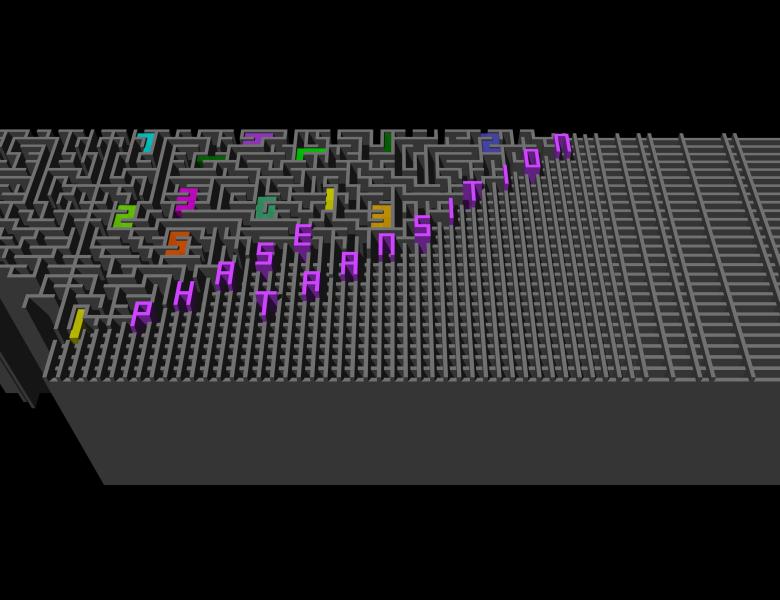
Abstract
We will discuss the role that subdominant states play in the design of algorithms for large-scale learning problems. We shall take as representative case the problem of learning random patterns with discrete synapses in feedforward networks. The standard statistical physics results show that this problem is exponentially dominated by metastable states and by isolated solutions that are extremely hard to find algorithmically.
A large deviation analysis based on a local entropy measure allows us to find analytical evidence for the existence of subdominant and extremely dense regions of solutions. We show numerically that these dense regions are surprisingly accessible by simple learning protocols. The large deviation measure introduced for the analytic study can be used as an objective function for a simple Monte Carlo Markov Chain which finds solutions efficiently.