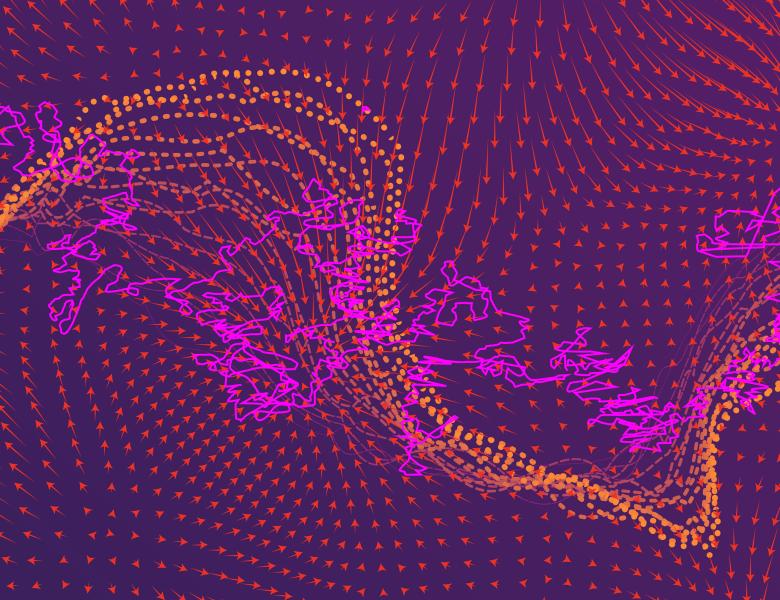
Abstract
Sampling and optimization are fundamental tasks in data science. While the literature on optimization for data science has developed widely in the past decade, with fine convergence rates for some methods, the literature on sampling remained mainly asymptotic until recently.
We study the proximal sampler introduced recently by Lee, Shen, and Tian. This sampling algorithm can be seen as a proximal point algorithm for the purpose of sampling. We will discuss the connection with the standard proximal point algorithm in optimization, and how the proximal sampler can be seen as an optimization algorithm over a space of probability measures.
Then, we will review an existing convergence guarantee relying on strong convexity, and show new convergence guarantees under weaker assumptions such as convexity and isoperimetry, which allow for nonconvexity. With these results, we obtain new state-of-the-art sampling guarantees for several classes of target distributions.