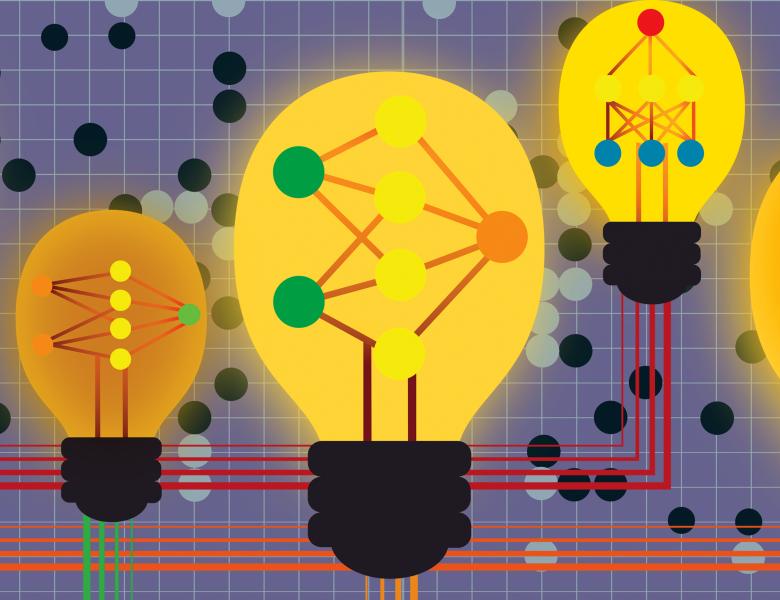
Abstract
Security game models have led to great success in modeling defender-attacker interaction or alike in security and environmental sustainability domains. Most of the earlier work in security games relies on mathematical programming-based algorithms to solve the game and compute the optimal strategy for the defender. However, for many problems that account for more practical aspects, the game model would be much more complex and mathematical programming-based methods are not applicable. In this talk, we introduce our work that leverages reinforcement learning to handle complex security games, including games with continuous action space, green security games with real-time information, and repeated games with unknown attacker types.