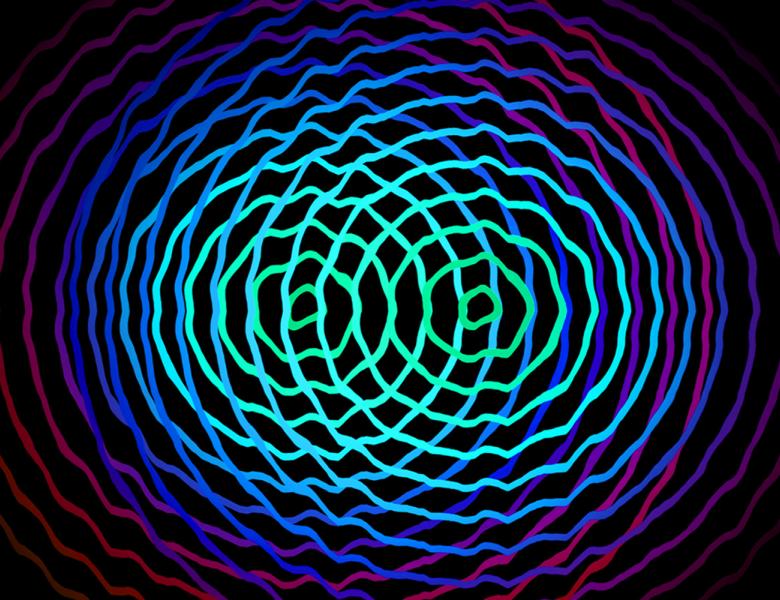
Abstract
1. Landscape Independence. Consider problems where instances come from a fixed distritution such as MAX CUT on d-regular random graphs of fixed size. Then regardless of the depth of the algorithm, for fixed parameters the expected value of cost funtion in the associated quantum state is independent of the instance up to finite size effects. This Landscape Independence shows that once good parameters have been chosen for one instance they can be used for others coming from the same distribution.
2.Sherrington Kirkpatrick. This is an all to all connected spin glass model. We have a method for calculating the expected value of the cost function at any parameters for typical instances in the infinite size limit. The method works for any depth p (independent of the number of spins) and numerically we have gone out to p of 8. I will also discuss provable concentration results which confirm Landscape Independence for the SK model.