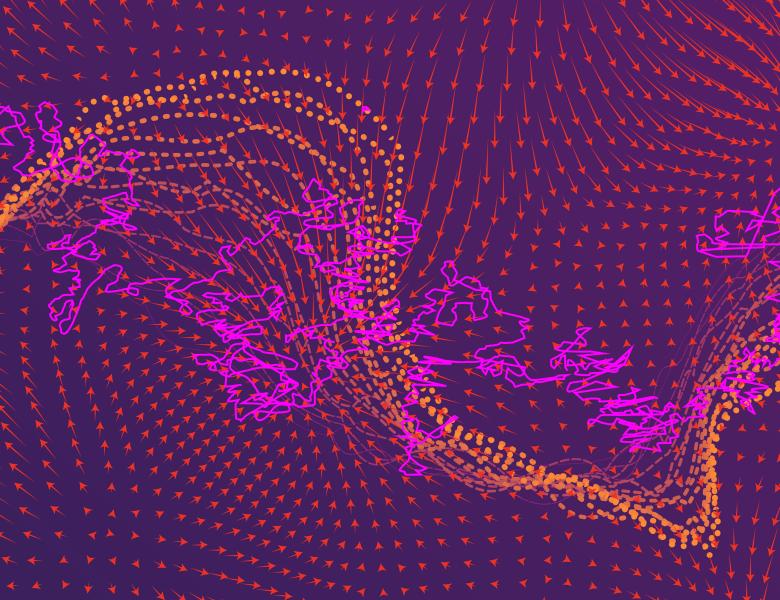
Abstract
We will discuss several interacting particle systems for sampling, or more precisely quantization of target measures. Namely instead of seeking an i.i.d. sample of the target measure we look to approximate the target probability distribution by a family of particles.
This can be cast as an optimization problem where the objective functional measures the dissimilarity to the target. This optimization can be addressed by approximating Wasserstein and related gradient flows. We will compare and contrast the Stein Variational Gradient Descent, projected gradient flows and gradient flows of Maximum Mean and Kernel Stein Discrepancy.
In practice, these are simulated by interacting particle systems, whose stationary states define an empirical measure approximating the target distribution. We investigate, theoretically and numerically, quantization properties of these approaches, i.e. how well is the target approximated by the empirical measure. In particular, we will discuss upper bounds on the quantization error of MMD and KSD with various kernels. The talk is based on joint work with Anna Korba and Lantian Xu.