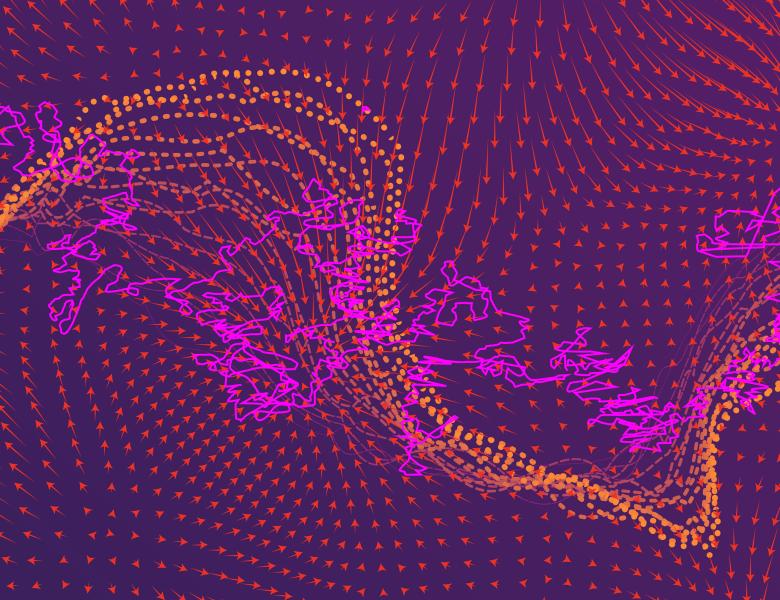
Abstract
Sampling from a complex distributionπand approximating its intractable normalizing constant $Z$ are challenging problems. In this talk, a novel family of importance samplers (IS) and Markov chain Monte Carlo (MCMC) samplers is derived. Given an invertible map $T$, these schemes combine (with weights) elements from the forward and backward orbits through points sampled from a proposal distribution $\rho$. The map $T$ does not leave the target $\pi$ invariant, hence the name NEO, standing for Non-Equilibrium Orbits. NEO-IS provides unbiased estimators of the normalizing constant and self-normalized IS estimators of expectations under $\pi$ while NEO-MCMC combines multiple NEO-IS estimates of the normalizing constant and an iterated sampling-importance resampling mechanism to sample from $\pi$.
Joint work with Achille Thin, Yazid Janati, Sylvain Le Corff, Charles Ollion, Arnaud Doucet, Eric Moulines, Christian Robert.