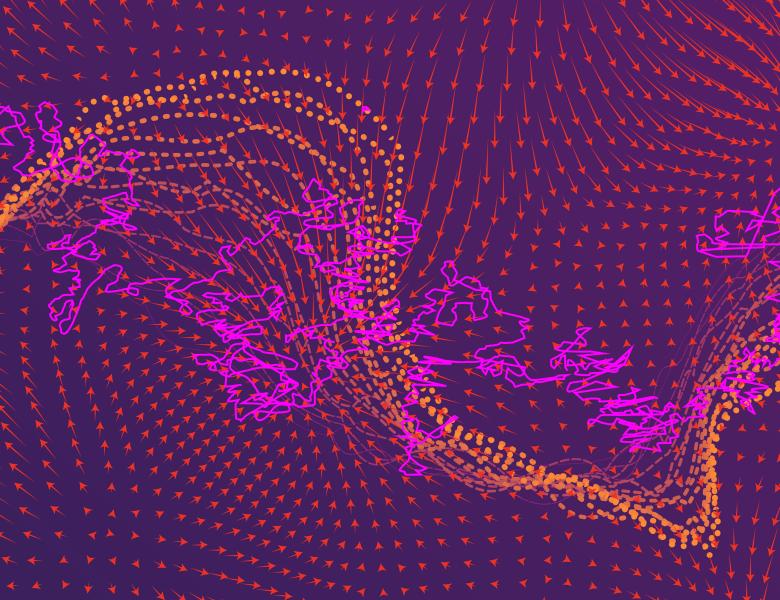
Abstract
The sampling problem to draw random samples from an unnormalized distribution plays an important role in many areas such as Bayesian inference and computational biology. In this work, we present new results on the proximal sampler, a recent method introduced by Lee, Shen, and Tian in 2021. The proximal sampler can be viewed as a sampling analog of the proximal point method in optimization and was proved to converge when the target distribution is strongly log-concave, assuming access to the restricted Gaussian oracle (RGO). We briefly discuss improved analysis of the proximal sampler that operates under weaker assumptions than previous works. We present an efficient implementation of the RGO when the target log density is neither convex nor smooth. Our implementation is accomplished using rejection sampling with a delicate proposal. Combining our RGO implementation and our improved convergence analysis we obtain a sampling algorithm that has state-of-the-art sampling guarantees in many settings, in particular, when the target distribution satisfies a functional inequality but is not necessarily smooth. This is joint work with Jiaming Liang, Sinho Chewi, Adil Salim, and Andre Wibisono.