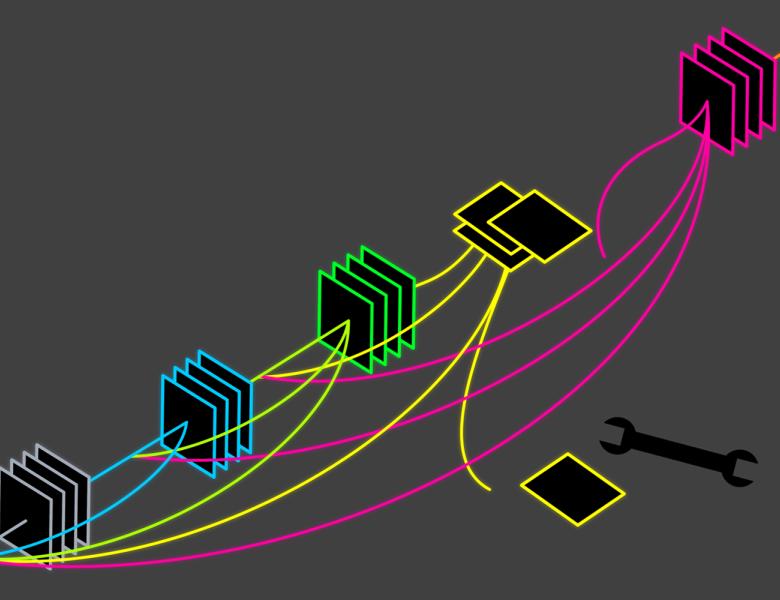
Abstract
A common paradigm for supervised learning is to learn linear predictors over a suitable representation either explicitly or using a kernel. One of the primary motivations for this work is to understand the limitations of such methods in the context of whether or not deep learning methods can go beyond.
The classic notions of dimensional and margin complexity aim to capture the power of such techniques, by providing sufficient conditions that ensure learnability with a linear/kernel representation. In this talk, we will define and study probabilistic variants of dimensional and margin complexity, which correspond to the minimal dimension or norm of an embedding required to approximately, rather than exactly, represent a given hypothesis class. These notions also turn out to be sufficient for learning using linear predictors or a kernel, but more importantly, some of these notions are also necessary. Thus they are better suited for discussing limitations of linear or kernel methods.
For regression problems, we prove general purpose lower bounds on these probabilistic notions, making connections to the statistical query model. For classification problems however, we identify a complexity theoretic barrier that makes the task of proving such explicit lower bounds highly non-trivial.
This talk is based on joint work with Omar Montasser and Nati Srebro [arXiv:2003.04180].