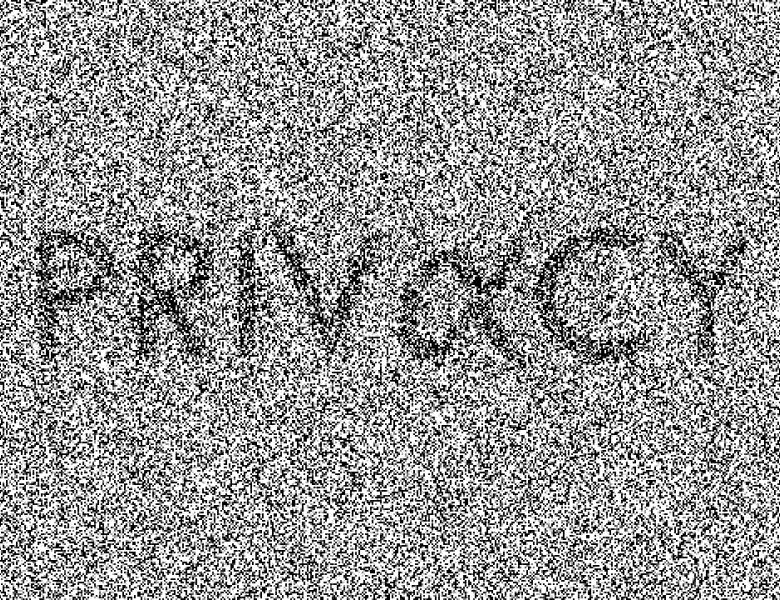
Abstract
The class of halfspaces forms an important primitive in machine learning as learning halfspaces implies learning many other concept classes. We study the question of privately learning halfspaces and present a private learner for halfspaces over an arbitrary finite domain X subset R^d with sample complexity poly(d,2^{\log^*|X|}). The building block for this learner is a differentially private algorithm for locating an approximate center point of points -- a high dimensional generalization of the median function. Our construction establishes a relationship between these two problems that is reminiscent of the relation between the median and learning one-dimensional thresholds [Bun et al. FOCS '15]. This relationship suggests that the problem of privately locating a center point may have further applications in the design of differentially private algorithms. We also provide a lower bound on the sample complexity for privately finding a point in the convex hull.