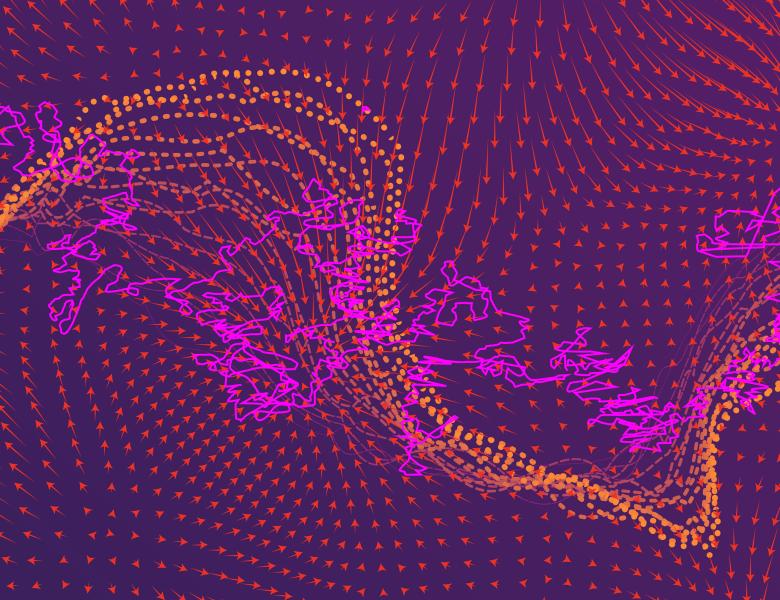
Abstract
Optimization and sampling are fundamental tasks of machine learning procedures. Although these two tasks seem unrelated, they can be unified through the lens of optimal transport theory. Indeed, sampling from some target distribution can be reformulated as minimizing a functional defined over a space of probability distributions called the Wasserstein space. In this talk, I will illustrate this connection in the setting of primal--dual optimization. Primal--dual optimization is a natural framework for minimizing composite functions over the Euclidean space. I will show how primal--dual optimization techniques can be carried over the Wasserstein space to sample from distributions whose log density is composite. In particular, by viewing it as a primal--dual algorithm, we will derive new results for the proximal Langevin algorithm in the nonsmooth setting.