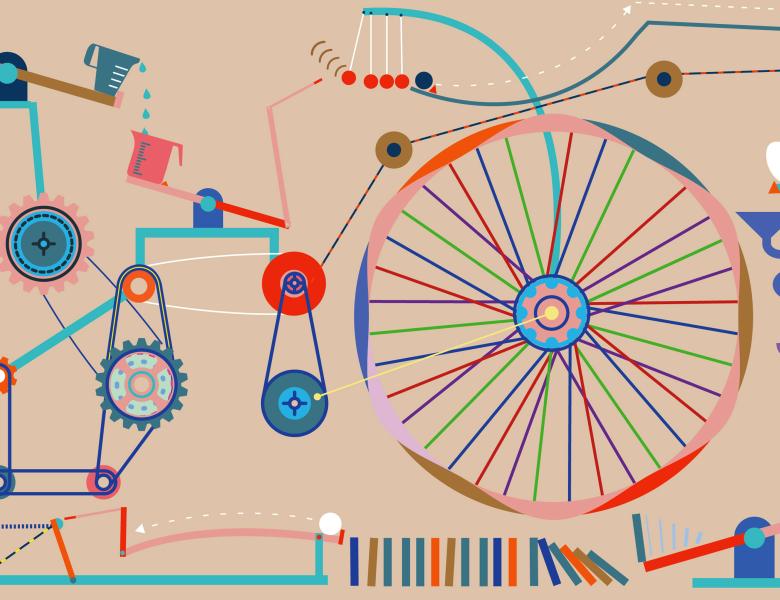
Abstract
Graphical models haven proven very beneficial for modeling classical and quantum information setups. This presentation consists of two parts. In the first part, we talk about graphical models for classical information processing. In particular, we show how certain polytopes associated with graphical models can be used to understand efficient, suboptimal algorithms like loopy belief propagation. (Such algorithms are, for example, used to decode low-density parity-check codes, a class of codes that appears in the recent 5G telecommunications standard.) In the second part, we talk about a class of graphical models suitable for quantum information processing. Certain polytopes and convex sets associated with these graphical models help to understand the difference between classical and quantum information processing, thereby generalizing Bell's theorem. Finally, we point out some surprising connections between the polytopes in the first part and the polytopes in the second part.