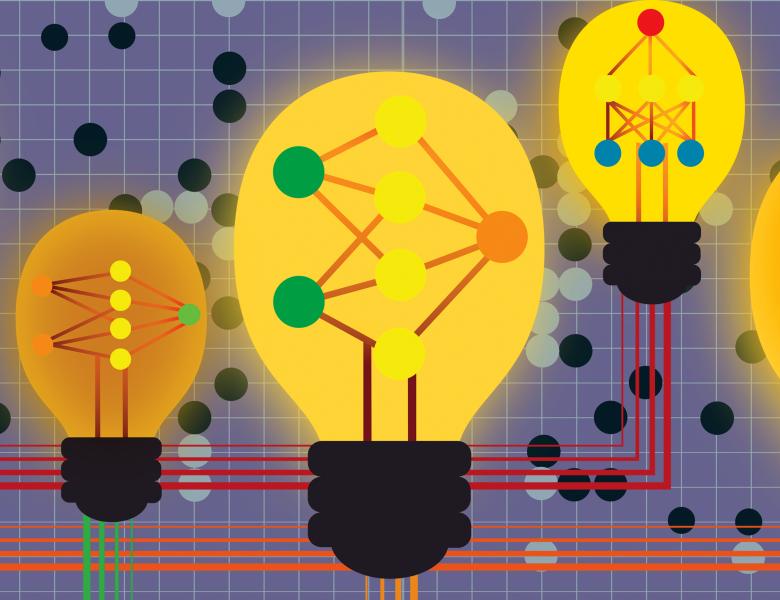
Abstract
Consider the estimation of policy gradient (PG) using data that maybe collected in off-policy manners. The talk presents a Policy Gradient Bellman Equation and shows how to leverage Q function approximator for policy gradient estimation via a double fitted iteration. We show that the double fitted iteration is equivalent to a plug-in estimator. Further, the PG estimation error bound is determined by a restricted chi-square divergence that quantifies the interplay between distribution shift and function approximation. A matching Cramer Rao lower bound is also provided.
Next, we show how to extend policy gradient method to reinforcement learning beyond cumulative rewards. In particular, consider the objective that is a general concave utility function of the state-action occupancy measure, containing as special cases maximal exploration, imitation and safety constrained RL. Such generality invalidates the Bellman equation and Sutton’s Policy Gradient Theorem. We derive a new Variational Policy Gradient Theorem for RL with general utilities, which establishes that the parametrized policy gradient may be obtained as the solution of a stochastic saddle point problem. We also exploit the hidden convexity of the Markov decision process and prove that the variational policy gradient scheme converges globally to the optimal policy for the general objective, though the optimization problem is nonconvex.