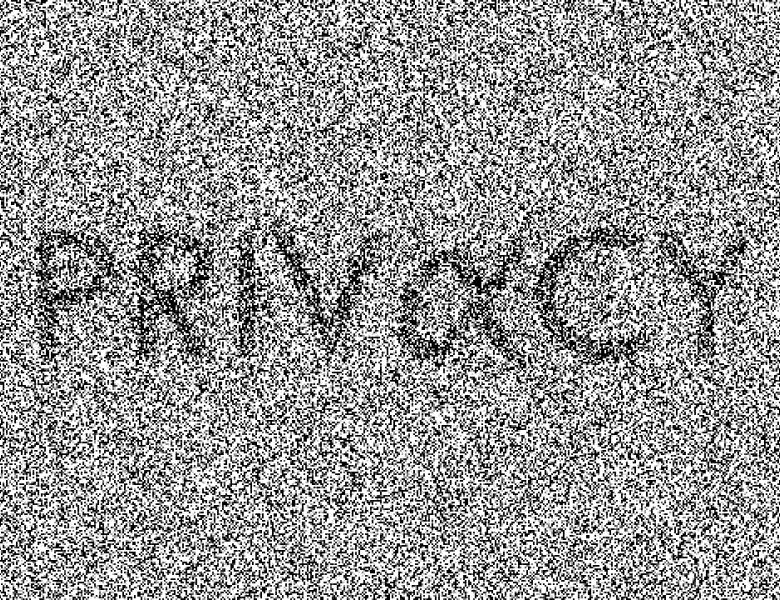
Abstract
We propose a method to release differentially private synthetic datasets using any parametric synthesizing model. Synthetic data, one of the popular methods in the disclosure control literature and among statistical agencies, releases alternative data values in place of the original ones. We guarantee ε-DP by sampling the parameters for the synthesizing distribution from the exponential mechanism, and we produce synthetic data that maximizes the distributional similarity of the synthetic data relative to the original data using a measure known as the pMSE. The flexibility of the framework allows for a variety of modeling choices given schematic or prior information. We also relax common DP assumptions concerning the distribution and boundedness of the original data, allowing for the release of differentially private data that is unbounded or continuous without additional assumptions. We prove theoretical results for the privacy guarantee, give simulation results for the accuracy of linear regression coefficients, and discuss two computational limitations.