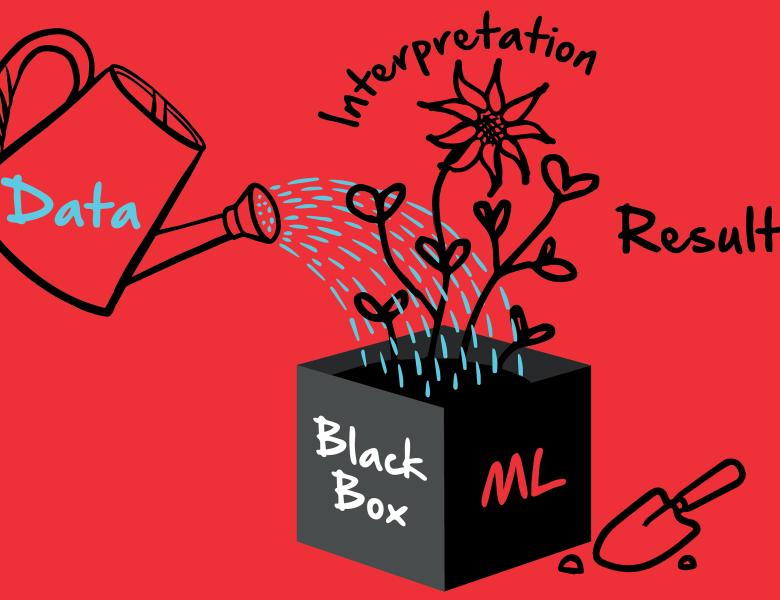
Abstract
Relevance estimators are algorithms used by social media platforms to determine what content is shown to users and its presentation order. These algorithms aim to personalize the platform's experience for users, increasing engagement and, therefore, platform revenue. However, many have concerns that the relevance estimation and personalization algorithms are opaque and can produce outcomes that are harmful to individuals or society. Legislations have been proposed in both the U.S. and the E.U. that mandate auditing of social media algorithms by external researchers. But auditing at scale risks disclosure of users' private data and platforms' proprietary algorithms, and thus far there has been no concrete technical proposal that can provide such auditing. We propose a new method for platform-supported auditing that can meet the goals of the proposed legislations