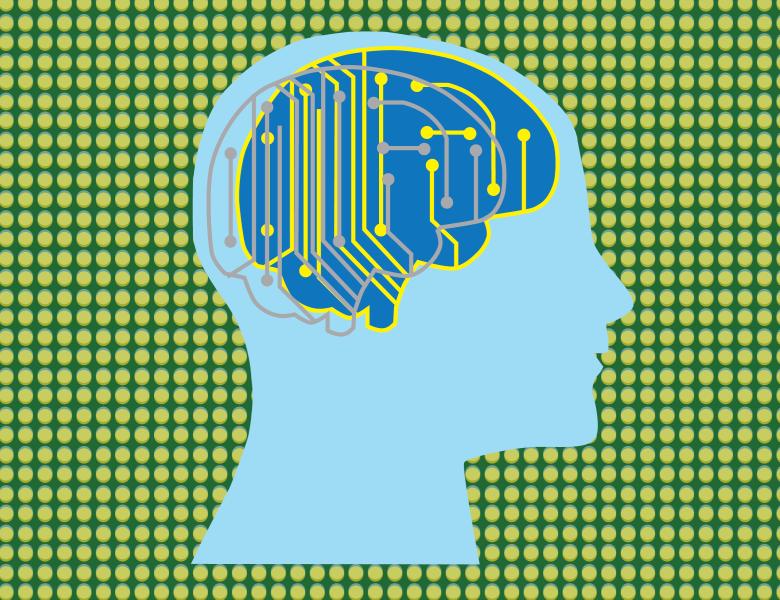
Abstract
Many forms of learning are guided by rewards and punishments. Humans and animals can be trained in complex tasks that consist of multiple epochs by the appropriate choice of reward contingencies. It is not well understood how association cortices learn to link sensory stimuli and memory representations to motor programs during reinforcement learning. Furthermore, there are many processing steps between the sensory cortices and the motor cortex. The learning rules for training such deep biological networks are only partially understood. I will outline a theory that explains how deep brain networks can learn a large variety of tasks when only stimuli and reward contingencies are varied, and present neurophysiological evidence in support of this theory.
The aim of the proposed architecture is to learn action values, i.e. the value of an action in a specific situation. I will demonstrate how networks can learn action values when they utilizes an ‘attentional’ feedback signal from motor cortex back to association cortex that “tags” synapses that should change to improve behavior. The resulting learning rule can train a simple neural network in many tasks that are in use in neurophysiology, including (1) delayed saccade tasks; (2) memory saccade tasks; (3) saccade-antisaccade tasks; (4) decision making tasks; and (5) classification tasks.
The proposed theory predicts that neurons at intermediate levels acquire visual responses and memory responses during training that resemble the tuning of neurons in association areas of the cerebral cortex of monkeys. The learning rule predicts that action values influence neuronal activity in sensory areas, as an attentional feedback effect. It is encouraging that insights from molecular, cellular and systems neuroscience can now be combined with insights from theories of reinforcement learning and deep artificial networks to develop a unified framework for learning in the brain.