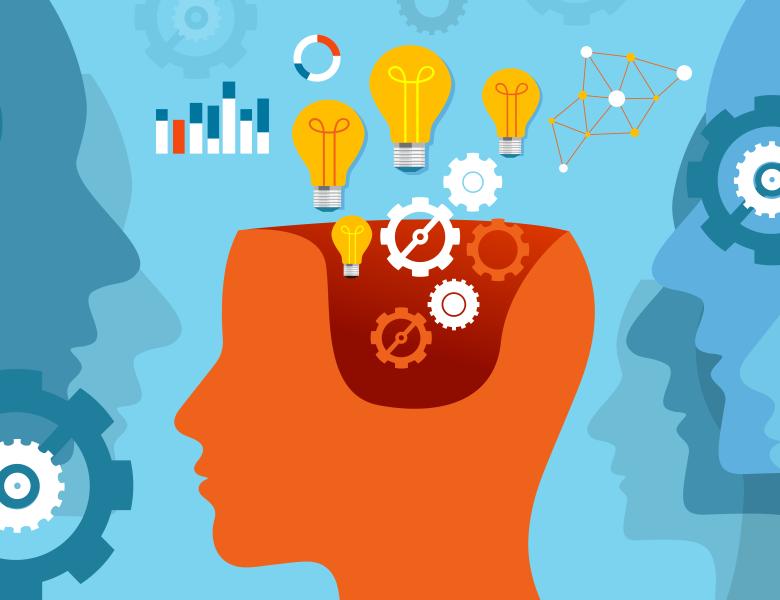
Abstract
Understanding the generalization properties of large, overparametrized, neural networks is a central problem in theoretical machine learning. Several insightful ideas have been proposed in this regard, among them: the implicit bias hypothesis, the possibility of having benign overfitting and the existence of feature learning regimes where neural networks learn the latent structure of data. However a precise understanding of the emergence/validity of these behaviors cannot be disentangled from the study of the non-linear training dynamics.
We use a technique from statistical physics, dynamical mean field theory, to study the training dynamics and obtain a rich picture of how generalization and overfitting arise in large overparametrized models. Joint work with Andrea Montanari.