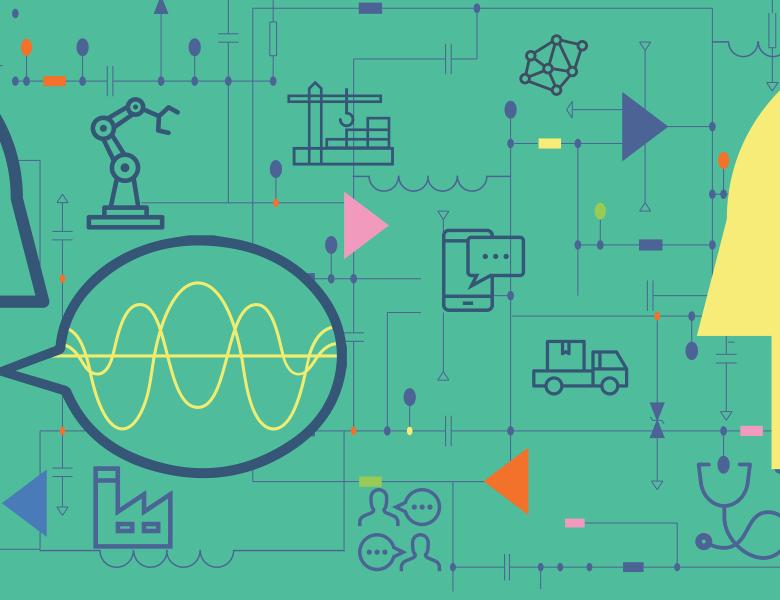
Abstract
Policy Gradient methods have been widely used empirically, and a number of theoretical works have studied their convergence and sample complexity properties in a local improvement setting. That is, when these methods are run starting from a favorable initial distribution that is suitably exploratory, there is a growing literature establishing the convergence properties for a number of policy gradient methods. However, global convergence guarantees without any further assumptions remain relatively limited, and have been obtained only recently even in the tabular setting.
In this talk, we introduce a novel policy gradient method called Policy Cover Policy Gradient (PC-PG), which active constructs a sequence of favorable initial distributions by designing exploratory policy covers that provide increasing coverage over the state space. Theoretically, we show that PC-PG finds a near optimal policy, assuming that the underlying MDP is a linear MDP in an infinite dimensional RKHS. We further show strong guarantees establishing robustness to model misspecification that go well beyond the standard \ell_\infty results, and show concrete consequences for an example of state aggregation. Empirically, we demonstrate the ease of combining our algorithm with standard approaches from deep learning, and show significant improvements on prior approaches in both reward-free and reward-driven settings.
[Joint work with Mikael Henaff, Sham Kakade and Wen Sun]