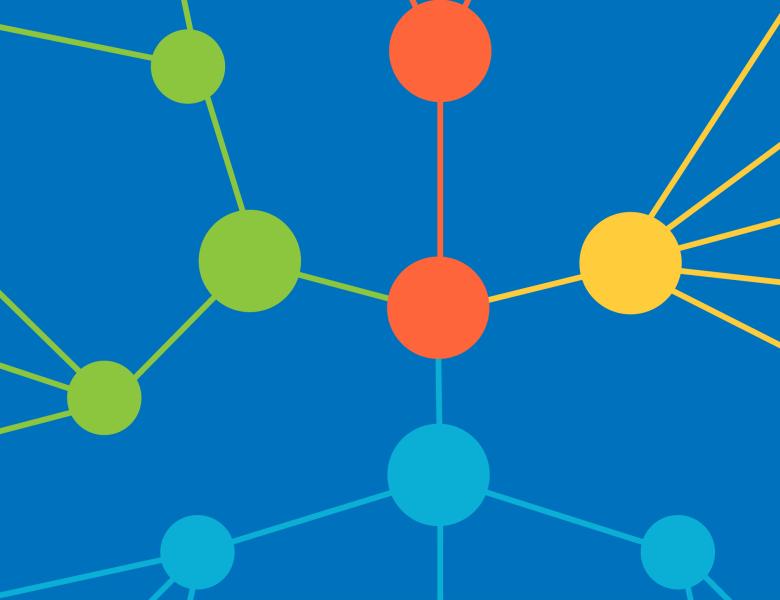
Abstract
Existing research on mechanistic interpretability usually tries to develop an informal human understanding of “how a model works,” making it hard to evaluate research results and raising concerns about scalability. Meanwhile formal proofs of model properties seem far out of reach both in theory and practice. In this talk I’ll discuss an alternative strategy for “explaining” a particular behavior of a given neural network. This notion is much weaker than proving that the network exhibits the behavior, but may still provide similar safety benefits. This talk will primarily motivate a research direction and a set of theoretical questions rather than presenting results.