Image
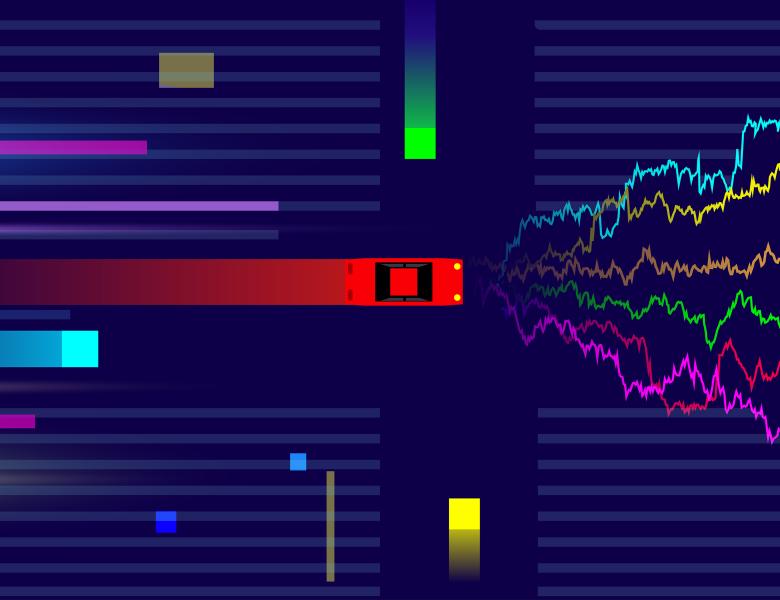
In this talk we will consider the number of predictions used by a learning-augmented algorithm as a resource measure. Focusing on two problems---online learning in the regret setting and online caching in the competitive-ratio setting---we show that a sublinear number of predictions suffices to achieve non-trivial performance gains.