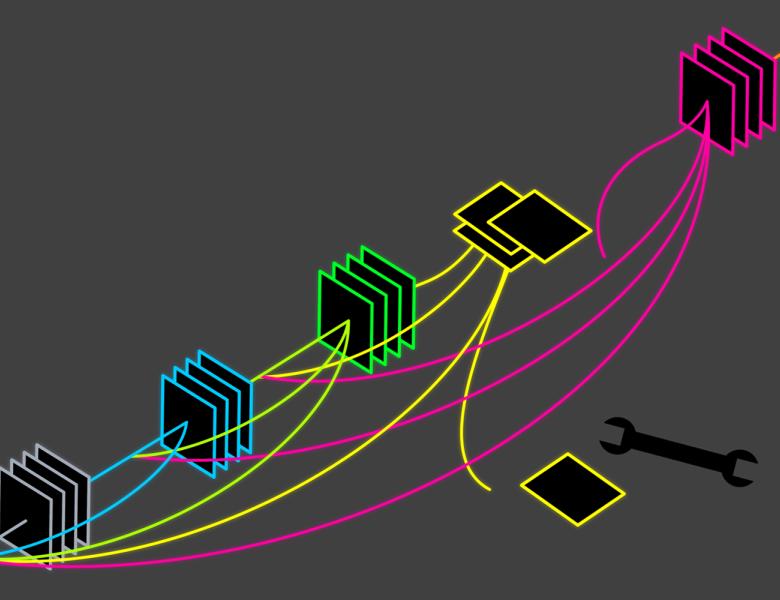
Abstract
Recently, a series of works have helped us understand why overparameterization can be harmless even when we interpolate all the training data. Essentially, having more features can have a regularizing effect that helps absorb the effects of noise in the training data (or model misspecification) without overly contaminating predictions. At the same time, as with any potentially strong regularizer, for learning to succeed we must ensure that the true signal in the data survives the inference process. In this talk, we take what we have learned from the regression setting to better understand overparameterized classification. By defining a simplified toy model (simultaneously related to the idea of spiked covariance models in statistics and low-pass filtering in signal processing) with a couple of natural knobs, we can see how there is a regime in which classification is fundamentally more forgiving than regression, though it can be understood using the same tools. The toy model also helps us better understand why simple naive perspectives on "margin maximization" cannot explain what is going on.
This is joint work with Misha Belkin, Daniel Hsu, and my students Vidya Muthukumar, Vignesh Subramanian, and Adhyyan Narang and is a direct consequence of the Simons Program last year.