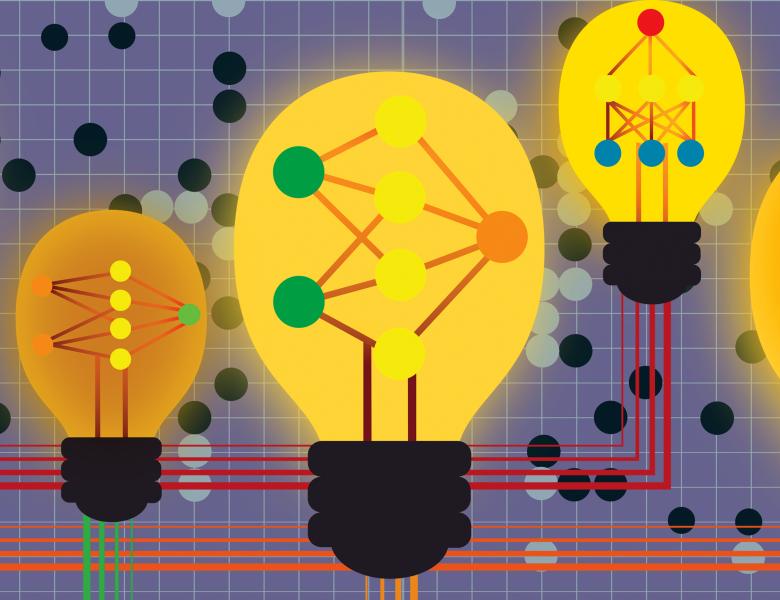
Abstract
Multi-agent interactions are increasingly important in the context of reinforcement learning and the theoretical foundations of policy gradient methods in this setting are largely elusive. In this work, we investigate the global convergence property of Natural Policy Gradient (NPG) algorithms in multi-agent learning. We first identify that vanilla NPG easily fails to converge in the policy parameter space, even in the single-agent setting, when the payoff is regularized by the entropy of the policy, which is the key to obtaining strong convergence guarantees as studied extensively in the recent literature. We then propose an optimistic variant of the NPG algorithm, for several standard multi-agent learning scenarios: two-player zero-sum matrix and Markov games, multi-player monotone games, and the corresponding settings with function approximation in policy parameterization. Contrast to several recent works on policy optimization in multi-agent learning, our algorithms are symmetric and decentralized, with global last-iterate convergence in the policy parameter space, and are able to handle large-scale games thanks to function approximation. Moreover, our results might also be of independent interest to solving nonconvex-nonconcave minimax optimization problems with certain structures.